Assessing regional healthcare service efficiency in China: a ten-year data envelopment analysis [2010–2019]
Highlight box
Key findings
• The study analyzed the efficiency of healthcare services across 31 provinces, municipalities, and autonomous regions in China from 2010 to 2019 using data envelopment analysis (DEA) and the Malmquist Productivity Index (MPI). Significant improvements in efficiency were observed in western and central China, with the eastern region nearly achieving optimal performance. Higher efficiency was observed in hospitals and coastal areas, highlighting regional disparities.
What is known and what is new?
• Hospital efficiency in China is generally high, though some regions have redundant medical resources, indicating a need for better resource allocation.
• This study offers a comprehensive, decade-long analysis of hospital efficiency, reflecting the impact of the 2009 healthcare reforms. It reveals evolving regional disparities and underscores the need for improved efficiency to enhance healthcare accessibility and equity.
What is the implication, and what should change now?
• Improving hospital efficiency is critical for enhancing healthcare accessibility and equity, especially in historically underserved regions. Enhanced efficiency in central and western regions can help reduce regional disparities in healthcare outcomes.
• There should be a targeted effort to allocate resources and implement best practices in regions with lower efficiency scores. Policymakers should continue to monitor efficiency trends and adjust healthcare reforms accordingly to ensure that improvements are sustained and equitable across all regions.
Introduction
The quest for equitable healthcare services is a universal concern, prominently so in the developing world where significant disparities exist. Addressing these inequalities has led researchers to evaluate current healthcare provisions and propose improvements (1,2). The operational efficiency of healthcare systems is crucial; it directly affects the quality and accessibility of medical services. Higher healthcare efficiency implies that the same amount of medical resources can provide services to a greater number of patients in need. An examination of Asian health systems revealed a general inefficiency, suggesting that better utilization of existing resources could enhance their performance (3). Similarly, only 58.33% of healthcare systems in Africa were deemed efficient (2), signalling a need for increased investment and more effective management practices in developing nations to improve healthcare outcomes. Comparative efforts are also observed in developed countries, emphasizing the universality of this challenge. A Japanese study highlighted that maintaining high-quality medical services is imperative, despite the economic pressures on hospital profitability. The proposed strategy involves identifying and incrementally addressing inefficiencies (4,5).
Investigations into healthcare system efficiency are essential for the advancement of medical service levels (3,6-8). China’s vast demographic and geogrfaphic scope, combined with historical economic disparities (6), presents unique challenges to its healthcare sector. To address this, the government has been progressively increasing healthcare expenditure, from a modest 6.6% of GDP in 2019 to an additional 1–1.5% in subsequent years (9). In 2009, the Chinese government implemented substantial healthcare reform measures aimed at improving the quality and accessibility of medical services (10). Improving hospital efficiency can enhance the accessibility of healthcare resources to some extent. Recent studies have focused on evaluating the efficiency of hospitals at provincial and national scales, identifying issues of inequality (11-15). Findings suggest a higher efficiency in premier hospitals and those located in coastal versus central regions (16). Moreover, the New Cooperative Medical System’s insurance reform has been associated with enhanced efficiency (17,18). Despite this, research predominantly concentrates on localized regions or specific sectors, such as operational efficiency or traditional Chinese medicine (11), with a dearth of comprehensive assessments on a national scale considering multifactorial efficiency impacts.
Data envelopment analysis (DEA) is an evaluative method that constructs a “frontier” to gauge the relative performance of entities, termed ‘decision-making units’ (DMUs), which may represent organizations or geographical regions (19,20). This technique has been broadly applied across diverse domains, encompassing both corporate and public sectors, including healthcare facilities (6,19). In this study, DEA was utilized to determine the scale and technological efficiencies of hospitals, which are indicative of the overall efficacy of healthcare service delivery (3). Furthermore, the Malmquist Productivity Index (MPI) was integrated to monitor the temporal progression of efficiency on an annual basis (1,8,21). These methodologies provided a detailed analysis of regional efficiency disparities and trends within the Chinese healthcare system throughout the defined evaluation period.
This study intends to fill this gap by analysing hospital efficiency across different Chinese regions over a decade. The goal is to delineate regional disparities and underlying causes, providing informed recommendations to aid the government’s ongoing reformative measures.
Methods
DEA
DEA established in 1978, represents a non-parametric approach to operational research. This method is distinguished by its ability to estimate the efficiency of DMUs—such as hospitals—without making assumptions about the functional form of the production process (19,20). Utilizing linear programming, DEA quantifies the efficiency and productivity of DMUs by comparing the observed input-output vectors, allowing for the simultaneous consideration of multiple inputs and outputs within the same model and timeframe (1,21). This study will calculate the efficiency of healthcare resource inputs and outputs across 31 provinces, municipalities, and autonomous regions in China to understand the level of healthcare efficiency in each region.
Efficiency in DEA is gauged by the proportional transformation of inputs or outputs, leading to the classification of models into two types: input-oriented and output-oriented. The input-oriented model aims to minimize inputs for a given level of outputs, while the output-oriented model seeks to maximize outputs without increasing inputs (18). The concept of efficiency measurement was initially underpinned by the assumption of constant returns to scale (CRS), suggesting that DMUs operate at an optimal scale (6,19,22). Later developments in 1984 introduced a variable returns to scale (VRS) model within DEA, facilitating the distinction between technical and scale efficiencies (19,20). The study of DEA efficiency involved utilizing models to calculate whether the inputs of a hospital are effectively translated into corresponding outputs, thereby assessing the overall efficiency.
Figure 1 illustrates the concept of economies of scale in the context of DEA, using a single input and a single output to compare five DMUs, labelled A through E. The figure delineates both CRS and VRS frontiers. Under the CRS assumption, only DMU C is deemed efficient. In contrast, when VRS is applied, DMUs A, C, D and E are all considered efficient. Scale efficiency (SE) is defined as the ratio of efficiency scores obtained under CRS to those obtained under VRS. SE serves as an indicator of whether a DMU is operating at an optimal scale.
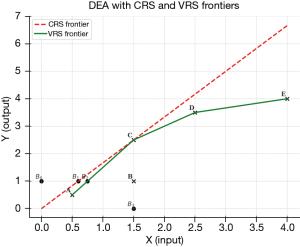
Efficiency is measured by the proximity of a DMU’s performance to the frontier; a DMU is fully efficient if its DEA score equals 1 and all slack variables are 0. A DEA score of 1 indicates that the DMU—in this case, a region—is operating on the efficiency frontier. This means it is using its inputs (e.g., resources like staff, funding, and infrastructure) in the most optimal way to produce the maximum possible outputs (e.g., patient services). A score less than 1 indicates that the DMU is not fully efficient. The further the score is from 1, the greater the inefficiency. The figure further quantifies the potential for enhancement, with the magnitude of slack signifying the scope for improvement in either inputs or outputs within the model’s orientation—input or output. A higher slack value suggests a greater potential for optimizing the corresponding factor (20). An ‘input-oriented’ approach is one where outputs remain constant, and the focus is on minimizing inputs to achieve these outputs. Conversely, an ‘output-oriented’ approach maintains inputs at their current levels while seeking to maximize outputs (1,19,23). For example, within an input-oriented CRS DEA model, the efficiency score of point B (denoted as θB, input, CRS) can be calculated using the ratio B0B1/B0B (in Figure 1). This ratio indicates the potential input reduction (1 − θB, input, CRS) that could yield the same level of output (19,20). Similarly, for the output-oriented CRS model, efficiency (θB, output, CRS) is represented by B3B/B3C, suggesting how output can be proportionally increased without changing the input level. The VRS model’s input-oriented efficiency for point B, θB, input, VRS, is defined by the ratio B0B2/B0B, which adjusts the input relative to the VRS frontier (19,20). Given the difficulty hospitals face in controlling outputs, many studies prefer the input-oriented model to gauge efficiency, as inputs are more manageable (6,20,21). This study adopts an input-oriented DEA model for this reason. CRS technical inefficiency can be further decomposed into two components: SE and ‘pure’ technical efficiency. In Figure 1, the segment B2B illustrates the ‘pure’ technical efficiency relative to the VRS model, while B1B indicates the same for the CRS model. The segment B1B2, therefore, represents SE (19). In practice, each hospital is characterized by multiple inputs and outputs. Efficiency is thus defined by the ratio of the aggregate of output values (total virtual output) to the aggregate of input values (total virtual input), with the optimization process determining the weights to maximize the unit’s performance score (1).
MPI
MPI, conceptualized by Malmquist and operationalized by Caves, Christensen, and Diewert, is a tool for assessing efficiency changes among various production entities (8,21). In this study, the MPI framework was utilized to examine the dynamic efficiency of the healthcare service system, frequently applied in measuring changes in total factor productivity (Tfpch). An MPI score greater than 1 indicates an enhancement in productivity, reflecting cost reductions and productivity gains.
Tfpch encompasses two dimensions: technical changes (Techch) and technical efficiency changes (Effch), with the latter further broken down into pure technical efficiency changes (Pech) and scale efficiency changes (Sech). Techch quantifies the influence of technological progress on production variations, where a score above 1 denotes beneficial technological advancements. Effch gauges the extent of efficiency improvement between two periods, with a score above 1 reflecting effective improvement. Pech assesses managerial efficiency, while Sech pertains to scale adjustments among DMUs. A Pech score below 1 suggests a need for enhanced management practices. Conversely, a Sech score below 1 signals the necessity for scale modifications to optimize efficiency (1,19).
Data collection
In evaluating healthcare service efficiency, selecting pertinent input and output variables is critical (1). This study’s data were sourced from the China Statistical Yearbooks spanning 2010–2019 (24). Capital and human resources are essential inputs to healthcare; hence, financial investments combined with the count of healthcare institutions and available beds constituted the capital input metric. The number of doctors and nurses were used as indicators of human resources input.
For outputs, the study measured service delivery using the average number of outpatient visits and annual hospitalization rates, specifically quantified as the number of outpatients (per hundred million) and inpatients (per ten thousand). Thirty-one provincial-level DMUs were classified into three geographical regions for analysis: eastern (12 provinces), western (10 provinces), and central (9 provinces).
The study’s subsequent analysis aimed to ascertain the efficiency of healthcare services in each province and to conduct comparative performance evaluations across the different regions. A decade’s worth of data [2010–2019] was compiled, incorporating five input indicators and two output indicators for each of the 31 provinces, municipalities, and autonomous regions under consideration.
Results
Data envelopment analysis
Table 1 and Figures 2-4 reveals the efficiency trends across eastern, central, and western regions over a decade, employing CRS, VRS, and SE models.
Table 1
Model | Year | |||||||||
---|---|---|---|---|---|---|---|---|---|---|
2010 | 2011 | 2012 | 2013 | 2014 | 2015 | 2016 | 2017 | 2018 | 2019 | |
CRS model | ||||||||||
East-area | 0.88 | 0.94 | 0.95 | 0.95 | 0.95 | 0.96 | 0.97 | 0.96 | 0.96 | 0.93 |
West-area | 0.78 | 0.92 | 0.93 | 0.92 | 0.93 | 0.94 | 0.95 | 0.94 | 0.94 | 0.92 |
Central area | 0.65 | 0.85 | 0.86 | 0.86 | 0.87 | 0.87 | 0.89 | 0.89 | 0.89 | 0.87 |
VRS model | ||||||||||
East-area | 0.92 | 0.97 | 0.98 | 0.98 | 0.98 | 0.98 | 0.99 | 0.98 | 0.98 | 0.97 |
West-area | 0.85 | 0.96 | 0.97 | 0.97 | 0.97 | 0.97 | 0.99 | 0.98 | 0.99 | 0.98 |
Central area | 0.68 | 0.87 | 0.87 | 0.88 | 0.89 | 0.89 | 0.90 | 0.91 | 0.91 | 0.90 |
Scale model | ||||||||||
East-area | 0.95 | 0.96 | 0.97 | 0.97 | 0.97 | 0.98 | 0.98 | 0.98 | 0.98 | 0.96 |
West-area | 0.91 | 0.95 | 0.96 | 0.95 | 0.96 | 0.96 | 0.96 | 0.96 | 0.95 | 0.94 |
Central area | 0.95 | 0.98 | 0.98 | 0.98 | 0.98 | 0.98 | 0.98 | 0.98 | 0.97 | 0.96 |
CRS, constant returns to scale; VRS, variable returns to scale.
In Figure 2, illustrating the CRS model, all regions’ efficiency scores were below 1, indicating room for improvement. From 2010 to 2011, there was a noticeable increase in efficiency scores across all regions, with a modest upward trend punctuated by fluctuations until 2016. This was followed by a marginal decline through to 2019. The central region’s efficiency was the lowest in 2010 at 0.64, improving to 0.85 in 2011, and reaching 0.87 by 2019. The western region consistently outperformed the central area, with efficiency values rising from 0.78 in 2010 to 0.93 in both 2011 and 2019. The eastern region exhibited the highest efficiency scores over the decade, slightly increasing from 0.87 in 2010 to 0.97 in 2019.
Figure 3 portrays the efficiency trends under the VRS model, echoing the pattern observed in the CRS model. Both eastern and western areas demonstrated stability post-2011, with efficiency scores hovering around 0.97. Conversely, the central area improved from a 2010 low of 0.64 to 0.87 in 2011, further climbing to 0.89 by 2019.
SE trends presented in Figure 4 were more subtle compared to the CRS and VRS models, with the decade’s scores for all regions oscillating between approximately 0.93 and 0.95. Notably, the western region recorded the lowest SE values among the three.
MPI
Table 2 presents the MPI assessments. The MPI analysis revealed that Effch scores were greater than or equal to 1 from the second to the seventh year, suggesting an efficiency improvement during this period, but dipped below 1 in the following three years. Techch demonstrated an upward trend with all annual values exceeding 1, except for the third year; however, a significant rise to 1.456 was observed in the final year. Pech maintained values above 1 throughout the decade, with the exception of the penultimate year, which registered slightly lower at 0.991. Sech began to fall below 1 from the seventh year onward. Tfpch recorded a value above 1 in the second year, contrasting with the first year. However, the third year saw a decline to 0.979, a trend that persisted until the ninth year. In the tenth year, there was a notable shift with the value increasing to 1.413. Over the entire period, the average scores for Effch (1.019) and Pech (1.021) indicated overall efficiency improvements, whereas the averages for Techch (0.977), Sech (0.999), and Tfpch (0.996) were marginally below the benchmark of 1.
Table 2
Year change | Efficiency change | Technical change | Pure efficiency change | Scale efficiency change | Total factor productivity changes |
---|---|---|---|---|---|
2 | 1.184 | 0.654 | 1.174 | 1.008 | 0.764 |
3 | 1.01 | 1.045 | 1.007 | 1.003 | 1.055 |
4 | 1 | 0.979 | 1.002 | 0.997 | 0.797 |
5 | 1.007 | 0.947 | 1.002 | 1.005 | 0.953 |
6 | 1.009 | 0.939 | 1.004 | 1.005 | 0.947 |
7 | 1.014 | 0.983 | 1.016 | 0.999 | 0.997 |
8 | 0.997 | 0.994 | 1 | 0.996 | 0.99 |
9 | 0.998 | 0.973 | 1.002 | 0.996 | 0.971 |
10 | 0.97 | 1.456 | 0.991 | 0.98 | 1.413 |
Mean | 1.019 | 0.977 | 1.021 | 0.999 | 0.996 |
According to Table 3, which summarizes the average MPI of the 31 provinces, municipalities, and autonomous regions across the decade, both Effch and Pech exceeded the value of 1. However, the averages for Techch, Sech, and Tfpch fell short of this threshold. Specifically, the Effch scores for seven provinces (Beijing, Jiangsu, Shandong, Guangdong, Sichuan, Yunnan, and Xizang) were below 1, suggesting efficiency losses. Nineteen provinces registered below 1 for Techch, indicating technological regression. On the Pech front, four provinces (Beijing, Jiangsu, Guangdong, and Yunnan) recorded means below 1, highlighting management inefficiencies. Sech scores for 11 provinces were below 1, signaling suboptimal scaling, and 12 provinces had Tfpch scores indicating a decrease in total factor productivity.
Table 3
Province-level administrative divisions | Efficiency change | Technical change | Pure efficiency change | Scale efficiency change | Total factor productivity changes |
---|---|---|---|---|---|
Liaoning | 1.066 | 0.924 | 1.067 | 1 | 0.985 |
Beijing | 0.996 | 0.843 | 0.997 | 0.999 | 0.84 |
Tianjin | 1.017 | 0.985 | 1.004 | 1.014 | 1.002 |
Hebei | 1.003 | 0.987 | 1.001 | 1.001 | 0.99 |
Shanghai | 1 | 1.012 | 1 | 1 | 1.012 |
Jiangsu | 0.997 | 1.026 | 0.997 | 1 | 1.023 |
Zhejiang | 1 | 0.999 | 1 | 1 | 0.999 |
Fujian | 1.019 | 0.993 | 1.019 | 1 | 1.012 |
Shandong | 0.961 | 0.968 | 1.002 | 0.959 | 0.93 |
Guangdong | 0.993 | 1.021 | 0.995 | 0.998 | 1.014 |
Hainan | 1.031 | 0.945 | 1 | 1.031 | 0.974 |
Guangxi | 1.015 | 0.996 | 1.02 | 0.996 | 1.011 |
Chongqing | 1.019 | 1.059 | 1.019 | 1 | 1.079 |
Sichuan | 0.998 | 0.974 | 1.005 | 0.992 | 0.972 |
Guizhou | 1.003 | 0.937 | 1.003 | 1 | 0.941 |
Yunnan | 0.991 | 1.033 | 0.989 | 1.002 | 1.024 |
Xizang | 0.969 | 0.835 | 1 | 0.969 | 0.809 |
Shannxi | 1.023 | 1.012 | 1.051 | 0.973 | 1.035 |
Gansu | 1.012 | 1.02 | 1.011 | 1.002 | 1.033 |
Qinghai | 1.04 | 0.934 | 1.046 | 0.995 | 0.972 |
Ningxia | 1.04 | 1.049 | 1 | 1.04 | 1.091 |
Xinjiang | 1.034 | 0.986 | 1.041 | 0.993 | 1.02 |
Jilin | 1.052 | 0.934 | 1.055 | 0.997 | 0.982 |
Heilongjiang | 1.085 | 0.962 | 1.09 | 0.996 | 1.044 |
Inner Mongolia | 1.06 | 1.006 | 1.059 | 1.002 | 1.067 |
Shanxi | 1.021 | 0.927 | 1.017 | 1.005 | 0.947 |
Anhui | 1.035 | 1.016 | 1.035 | 1 | 1.052 |
Jiangxi | 1.035 | 1.005 | 1.034 | 1 | 1.04 |
Henan | 1.006 | 1.002 | 1.004 | 1.001 | 1.008 |
Hubei | 1.037 | 0.973 | 1.035 | 1.002 | 1.009 |
Hunan | 1.052 | 0.966 | 1.052 | 1 | 1.017 |
The input slack
Figures 5-9 detail the input slack values across 31 Chinese provinces over a decade, offering insights into resource utilization within the healthcare sector.
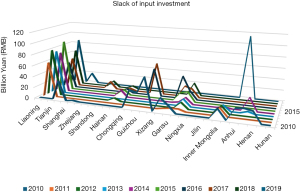
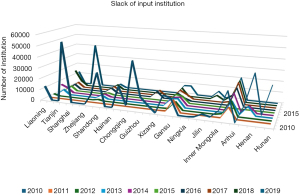
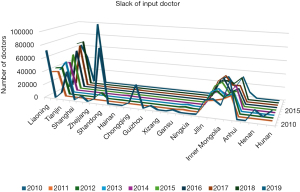
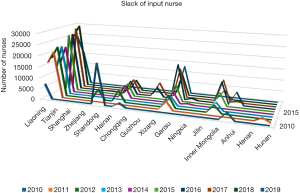
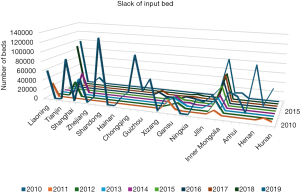
In 2010, notable slack in financial investment (in Figure 5) was observed in Hebei and several western (Chongqing, Sichuan, Guizhou, Yunnan) and central provinces (Inner Mongolia, Anhui, Jiangxi, Henan), with Yunnan exhibiting the most significant slack at approximately 50. Throughout the decade, Liaoning and Beijing consistently displayed slack values ranging from 40 to 85, with Jiangxi registering the highest slack in 2019 at 113.
As for healthcare institutions (in Figure 6), 10 provinces had zero slack in 2010. Hebei presented the largest slack at 46,000, a value which remained elevated, reaching 40,000 in 2019. Gansu and Shanxi experienced persistent slack values of around 8,000 and 20,000, respectively, across the years.
Doctor slack in 2010 was widespread, reducing in subsequent years (in Figure 7). Liaoning and Beijing maintained slack values between 35,000 and 70,000 for 9 years. Jilin, Heilongjiang, Inner Mongolia, and Shanxi consistently showed approximately 25,000 slack. Anhui’s slack in 2019 was noted at 8,000.
For nursing input (in Figure 8), Liaoning and Beijing were prominent, with slack values around 20,000 for 9 years. Shanxi’s slack increased from 2,500 in 2011 to 12,500 in 2019, with an apex of 13,000 in 2016. Guangdong’s slack fluctuated around 4,000 for 6 years, dropping to zero in 2019, a trend mirrored by Heilongjiang.
Regarding hospital beds (in Figure 9), a significant slack was present in various provinces in 2010, except for nine provinces that showed zero slack. Shandong had the highest slack at nearly 100,000. Heilongjiang’s slack escalated from 10,000 in 2010 to 80,000 in 2019. In the final year, Heilongjiang and Liaoning recorded the highest slack values at 80,000 and 90,000, respectively, indicative of substantial room for improvement in hospital bed management.
Discussion
The observed surge in healthcare efficiencies across all three examined regions from 2010 to 2011, as presented in Table 1 and Figures 2-4, aligns with the period of intensive healthcare reforms initiated by the government. These reforms aimed to substantially improve the service level of healthcare institutions. Post-2011, efficiencies in these areas plateaued, maintaining a steady state through 2019, corroborating findings by Chen et al. (25).
The western and eastern regions of China sustained high comprehensive efficiencies, with scores nearing the optimal 1.0, reflective of the abundant resources in the rapidly developing eastern region that satisfy healthcare demands. This contrasts with the central region, which, despite possessing adequate inputs, did not achieve similar efficiency gains. This discrepancy suggests an underutilization of resources and indicates that local healthcare services may not be optimally tailored to community needs (16).
The central region’s lower technical efficiency implies that technological advancements have not been effectively integrated into health service delivery. This may be partly due to the region’s difficulty in attracting and retaining skilled professionals who can leverage new technologies. The notion of SE, which remained comparable across all regions, is indicative of the government’s substantial investment, equipping healthcare institutions with sufficient infrastructure to serve the populace (17,26).
MPI provides insights into the dynamic efficiency changes across various healthcare models. The Effch component of MPI, particularly relevant in gauging the degree of technical innovation over two periods, reflects the effectiveness of recent healthcare reforms. From 2010 to 2016, the Effch MPI exceeded 1 across all regions, signifying that innovations played a vital role in enhancing healthcare services.
Although new healthcare technologies have been steadily introduced over the past decade, it was not until 2019 that a significant leap in technical efficiency was observed. This aligns with the increased integration of internet utilities and artificial intelligence in healthcare, which has been instrumental in boosting service efficiency and quality. The Pech values, surpassing 1 for all years except 2019, suggest that management practices effectively adapted to fulfill evolving needs.
The Sech mean for the decade stood at 0.999, indicating that the existing scale of healthcare institutions was well-aligned with the demand, thus negating the need for further adjustments. Post-reform government investments have evidently catalysed positive changes, as indicated by the improvements in SE (27,28).
Tfpch, which encapsulates the overall efficiency level, showed a marked increase to 1.413 in 2019, suggesting that concerted efforts by healthcare professionals and the government to streamline operations and reduce costs have borne fruit. As living standards rise, public health consciousness grows, escalating the demand for healthcare services. This burgeoning demand necessitates continued efforts to amplify healthcare efficiency, ensuring that healthcare systems are well-equipped to meet the future needs of society (25,29).
MPI analysis at the provincial level indicates that Effch and Pech scores exceeded the unity threshold for seven provinces. This suggests enhanced service delivery in these regions, though the overall efficiency gain was not uniform. Notably, all central provinces showed gains in efficiency, while select eastern and western provinces did not.
Beijing, Jiangsu, Guangdong, and Yunnan recorded Pech values below one. Beijing, Jiangsu, and Guangdong, as economically advanced provinces, display superior management capabilities yet exhibit potential for further refinement. Conversely, Yunnan’s service improvements have not translated into higher efficiency, likely due to the region’s economic challenges.
The Sech values were narrowly below one, implying that the current scale of healthcare institutions adequately meets the service requirements and populace needs. Interestingly, the Techch average surpassed one in half of the Chinese provinces, with notable progress in nine western and central provinces. This reflects how the eastern region’s stronger economic and technological infrastructure aids in narrowing efficiency gaps and bolstering service efficiency through the adoption of new technologies.
Tfpch presented an encouraging picture, with most provinces showing efficiency values above one, averaging near the benchmark of 0.996. However, Beijing exhibited a consistent shortfall across all five MPI models. This anomaly might be attributed to Beijing’s substantial financial resources and talent pool, which paradoxically results in resource redundancy and inefficiencies (10,30).
Figures 5-9 reveal that since the medical reforms initiated in 2011, certain regions in China have exhibited improvements across various healthcare indicators. Notably, Beijing, as the capital and a developed region, has an abundance of healthcare resources. However, the data suggests a need for the capital to rationalize its resource allocation, reducing excess in investment, and workforce including doctors and nurses, to avoid redundancy.
In the western provinces of Shannxi and Gansu, there is a call to re-evaluate the number of healthcare institutions and beds. Despite these provinces’ expansive land, their populations do not justify the current provision levels. This mismatch suggests an opportunity to optimize healthcare resources to better suit the demographic demands.
The industrial provinces of Jilin, Heilongjiang, and Liaoning are advised to reconsider the scale of their healthcare institutions and staffing levels. The observed decline in heavy industries and the pivot towards greener energy alternatives have led to demographic shifts, with populations migrating in search of new employment opportunities. This transition has resulted in an oversupply of healthcare resources relative to the decreasing population, indicating a need for realignment (19,29).
While DEA is a robust tool commonly applied in assessing the efficiency of healthcare institutions, it does have its constraints. One significant limitation of the DEA approach is its inability to account for management quality and environmental factors that could significantly influence efficiency. Additionally, DEA does not readily allow for the disaggregation of factors into subgroups for a more granular analysis of efficiency-related issues.
Therefore, while this study provides a macro-level assessment of efficiency across regions, it does not delve into the nuanced, province-specific scenarios that may offer more detailed insights. Subsequent studies should aim to refine the methodology to incorporate these influential variables and provide a more comprehensive analysis. Further research is also necessary to dissect the contributory factors of efficiency at a more localized level within each province, enhancing the applicability of the findings to targeted improvement strategies.
Conclusions
The study has demonstrated that healthcare efficiency has notably improved in China’s western and central regions following medical reforms. The reforms have effectively redistributed health resources to these areas, thereby addressing the needs of the population and mitigating the disparities in health service allocation that are often associated with uneven economic development. The advancements have also opened pathways for the utilization of advanced technologies in poorer regions, contributing to a rise in healthcare service levels.
The equitable distribution of professional staff across all three regions is indicative of a commitment to equality in healthcare provision. Notably, the management of healthcare institutions in China has consistently demonstrated a high level of competence.
Distinct from many studies that limit their scope to a single year or location, this investigation extends over a decade and encompasses all 31 provinces, municipalities, and autonomous regions, offering a comprehensive, longitudinal view of the efficiency and equality of healthcare services in China.
Acknowledgments
Funding: None.
Footnote
Peer Review File: Available at https://jhmhp.amegroups.com/article/view/10.21037/jhmhp-24-63/prf
Conflicts of Interest: Both authors have completed the ICMJE uniform disclosure form (available at https://jhmhp.amegroups.com/article/view/10.21037/jhmhp-24-63/coif). The authors have no conflicts of interest to declare.
Ethical Statement: The authors are accountable for all aspects of the work in ensuring that questions related to the accuracy or integrity of any part of the work are appropriately investigated and resolved.
Open Access Statement: This is an Open Access article distributed in accordance with the Creative Commons Attribution-NonCommercial-NoDerivs 4.0 International License (CC BY-NC-ND 4.0), which permits the non-commercial replication and distribution of the article with the strict proviso that no changes or edits are made and the original work is properly cited (including links to both the formal publication through the relevant DOI and the license). See: https://creativecommons.org/licenses/by-nc-nd/4.0/.
References
- Alatawi AD, Niessen LW, Khan JAM. Efficiency evaluation of public hospitals in Saudi Arabia: an application of data envelopment analysis. BMJ Open 2020;10:e031924. [Crossref] [PubMed]
- Top M, Konca M, Sapaz B. Technical efficiency of healthcare systems in African countries: An application based on data envelopment analysis. Health Policy Technol 2020;9:62-8. [Crossref]
- Nayar P, Ozcan YA. Data envelopment analysis comparison of hospital efficiency and quality. J Med Syst 2008;32:193-9. [Crossref] [PubMed]
- Kawaguchi H, Tone K, Tsutsui M. Estimation of the efficiency of Japanese hospitals using a dynamic and network data envelopment analysis model. Health Care Manag Sci 2014;17:101-12. [Crossref] [PubMed]
- Sasaki K, Ishikawa T, Fujiwara K, et al. Measuring Municipality Hospitals Efficiency in Hokkaido by using an Assurance Region Model. Med Inform 2017;37:81-6.
- Pelone F, Kringos DS, Romaniello A, et al. Primary care efficiency measurement using data envelopment analysis: a systematic review. J Med Syst 2015;39:156. [Crossref] [PubMed]
- Zubir MZ, Noor AA, Mohd Rizal AM, et al. Approach in inputs & outputs selection of Data Envelopment Analysis (DEA) efficiency measurement in hospitals: A systematic review. PLoS One 2024;19:e0293694. [Crossref] [PubMed]
- Bağcı H, Çil Koçyiğit S. Evaluating the decentralization of public hospitals in Turkey in terms of technical efficiency: data envelopment analysis and Malmquist index. Benchmarking 2023;30:4425-60. [Crossref]
- Hu HH, Qi Q, Yang CH. Analysis of hospital technical efficiency in China: Effect of health insurance reform. China Economic Review 2012;23:865-77.
- The State Council of the People's Republic of China. Implementation Plan for the Recent Priorities of the Health Care System Reform (2009–2011). 2009. Available online: https://www.gov.cn/zwgk/2009-04/07/content_1279256.htm
- Li Z, Si X, Ding Z, et al. Measurement and Evaluation of the Operating Efficiency of China's Basic Pension Insurance: Based on Three-Stage DEA Model. Risk Manag Healthc Policy 2021;14:3333-48. [Crossref] [PubMed]
- Liu X, Jia C, Cheng J, et al. Measuring Productivity and Relative Efficiency of Public Tertiary Traditional Chinese Medicine Hospitals in Hubei, China. Inquiry 2023;60:469580231190576. [Crossref] [PubMed]
- Sun M, Ye Y, Zhang G, et al. Measuring the efficiency of public hospitals: A multistage data envelopment analysis in Fujian Province, China. Front Public Health 2023;11:1091811. [Crossref] [PubMed]
- Zhang CW, Yang Y. Appraisal of regional hospital efficiency and healthcare quality in China: Impacts of subsidies and marketization. Socio-Economic Planning Sciences 2023;89:101695. [Crossref]
- Zhou J, Peng R, Chang Y, et al. Analyzing the efficiency of Chinese primary healthcare institutions using the Malmquist-DEA approach: Evidence from urban and rural areas. Front Public Health 2023;11:1073552. [Crossref] [PubMed]
- Hu HH, Qi Q, Yang CH. Evaluation of China's regional hospital efficiency: DEA approach with undesirable output. J Oper Res Soc 2012;63:715-25. [Crossref]
- Zhao J, Yang Y, Ogasawara K. Measuring the Inequalities in the Distribution of Public Healthcare Resources by the HRDI (Health Resources Density Index): Data Analysis from 2010 to 2019. Healthcare (Basel) 2022;10:1401. [Crossref] [PubMed]
- Li Q, Wei J, Jiang F, et al. Equity and efficiency of health care resource allocation in Jiangsu Province, China. Int J Equity Health 2020;19:211. [Crossref] [PubMed]
- Cooper WW, Seiford LM, Zhu J. Handbook on data envelopment analysis. New York, NY: Springer; 2011.
- Cooper WW, Seiford LM, Tone K. Introduction to data envelopment analysis and its uses: with DEA-solver software and references. New York, NY: Springer; 2006.
- Kohl S, Schoenfelder J, Fügener A, et al. The use of Data Envelopment Analysis (DEA) in healthcare with a focus on hospitals. Health Care Manag Sci 2019;22:245-86. [Crossref] [PubMed]
- Cooper WW, Seiford LM, Tone K. Data envelopment analysis: a comprehensive text with models, applications, references and DEA-solver software. New York, NY: Springer; 2007.
- Dong S, Zuo Y, Guo S, et al. Data envelopment analysis for relative efficiency measurement of Chinese hospitals: a systematic review. Res Health Sci 2017;2:79-103. [Crossref]
- National Bureau of Statistics of China. China Statistical Yearbook. 2010-2019. Available online: https://www.stats.gov.cn/english/Statisticaldata/yearbook/
- Chen Z, Chen X, Gan X, et al. Technical Efficiency of Regional Public Hospitals in China Based on the Three-Stage DEA. Int J Environ Res Public Health 2020;17:9383. [Crossref] [PubMed]
- Eggleston K, Ling L, Qingyue M, et al. Health service delivery in China: a literature review. Health Econ 2008;17:149-65. [Crossref] [PubMed]
- The State Council of the People's Republic of China. The Notice of the General Office of the State Council on Issuing the Outline for the Planning of the National Medical and Health Service System (2015–2020). 2015. Available online: https://www.gov.cn/zhengce/content/2015-03/30/content_9560.htm
- National Health Commission of the People's Republic of China. Guiding Opinions of the General Office of the State Council on Establishing a Modern Hospital Management System. 2017. Available online: http://www.nhc.gov.cn/wjw/gwywj/201707/5b750f71082b48dca63779d4922b7aaf.shtml
- Yip WC, Hsiao W, Meng Q, et al. Realignment of incentives for health-care providers in China. Lancet 2010;375:1120-30. [Crossref] [PubMed]
- Li H, Dong S, Liu T. Relative efficiency and productivity: a preliminary exploration of public hospitals in Beijing, China. BMC Health Serv Res 2014;14:158. [Crossref] [PubMed]
Cite this article as: Zhao J, Ogasawara K. Assessing regional healthcare service efficiency in China: a ten-year data envelopment analysis [2010–2019]. J Hosp Manag Health Policy 2024;8:23.