Impact of heatwaves, demographics, and environment on hospitalization rates in Paris
Highlight box
Key findings
• Notable findings from our study reveal the intricate interplay between heatwaves, demographics, and health outcomes in Paris.
What is known and what is new?
• While the health risks of heatwaves are established, our research provides fresh insights into the combined impact of demographic factors and architectural attributes on hospitalization rates.
• Our manuscript contributes by comprehensively assessing heatwave effects on hospitalizations across diverse health conditions in Paris. We highlight demographic and environmental variables as key determinants during extreme climatic events.
What is the implication, and what should change now?
• Our findings emphasize the urgency for targeted interventions. Health authorities should focus on vulnerable groups, especially the elderly. Urban planning should incorporate air conditioning and elevators, and healthcare strategies must address conditions sensitive to heatwaves.
• In conclusion, our study underscores the necessity of a holistic approach to public health amidst climate change. Tailored interventions for urban settings like Paris can effectively mitigate heatwave-related health risks and ensure population well-being.
Introduction
Background
Climate change is increasingly recognized as a multi-faceted global emergency with wide-ranging repercussions, not least of which is its profound impact on human health (1). Among the various settings affected, urban environments are particularly vulnerable due to their density and infrastructure. Paris offers a compelling case study in this context. Characterized by a modified oceanic climate, the city experiences temperature fluctuations that can reach extreme levels, thereby amplifying specific health risks such as dehydration, heatstroke, and cardiovascular complications. These adverse health effects are corroborated by a growing body of global data, reinforcing the urgency of addressing climatic shifts as a public health imperative (2).
Heatwaves, a direct consequence of global warming, as documented in (https://www.ipcc.ch/report/ar6/wg1/downloads/report/IPCC_AR6_WGI_Chapter11.pdf), have been scientifically linked to a surge in morbidity and mortality rates (3,4). A global review by Campbell et al. identified extensive research on the health impacts of heatwaves in 854 locations, pinpointing the major toll on health systems, especially ambulance and hospital workloads (5). Furthermore, in China, a country characterized by its diverse climatic conditions, a comprehensive study encompassing 272 cities revealed that heatwaves are associated with statistically significant increases in mortality. These impacts were particularly pronounced among vulnerable groups such as the elderly, females, and individuals with lower levels of education. (6).
Recent research has gone beyond merely identifying these health risks to quantifying the economic toll they take on healthcare systems. These studies reveal that the economic burden, including healthcare costs and productivity losses, can be staggering (4). Additionally, demographic analyses indicate that elderly populations are at a heightened risk of heat-related hospital admissions, thereby spotlighting the role of demographic factors in heat vulnerability (7).
In February 2020, National School of Statistics and Economic Administration (INSEE) issued a report that served as a wake-up call, revealing that France had already crossed alarming temperature thresholds, with peaks reaching up to 40 degrees Celsius (8). However, despite growing awareness and an increasing sense of urgency, there exists a significant research gap. Current studies often fall short of integrating multiple variables—such as heatwaves, environmental factors, and demographic elements—to assess their cumulative impact on healthcare needs, especially in densely populated urban locales like Paris (3).
Rationale and knowledge gap
The growing frequency and intensity of heatwaves serve as major risk factors for public health, manifesting in increased rates of hospitalizations and a range of heat-sensitive ailments, including heat exhaustion, heat cramps, and more severe heat-related illnesses (3). While INSEE’s report has served as a critical indicator of the looming health risks, specifically highlighting the issue in the French context (8), the research landscape remains fragmented. There is a conspicuous absence of comprehensive, integrative research that combines climatic phenomena with other vital environmental and demographic variables (4,7).
Moreover, the issue extends beyond individual health and healthcare costs. Vulnerable groups, such as the elderly, children, and those with pre-existing health conditions, are disproportionately affected (7). At the European level, projections related to temperature-induced mortality are disconcerting, signaling an urgent need for targeted interventions (9). Furthermore, a growing body of evidence suggests that the impact of temperature fluctuations extends beyond mortality to affect a broad spectrum of health conditions, including respiratory and cardiovascular diseases (10).
Objectives
Given the complex interplay between climate change and public health (10), this study aims to address the existing academic gap by offering a nuanced examination of the synergistic effects of heatwaves, environmental factors, and demographic variables on healthcare needs in Paris. Specifically, the study will delve into hospitalization rates as a key indicator of healthcare burden. It will explore how extreme weather conditions interact with other variables such as age demographics, building infrastructure, and the prevalence of air conditioning systems to influence health outcomes among Parisian residents. We present this article in accordance with the STROBE reporting checklist (available at https://jhmhp.amegroups.com/article/view/10.21037/jhmhp-23-115/rc).
Methods
Study population
In our research, we employed a comprehensive healthcare data approach, drawing from vital sources like the National Health Data System (SNDS) and the Technical Agency for Information on Hospitalization (ATIH). Our primary focus centered on extracting insights from the Program for Medicalization of Information Systems (PMSI) tables, providing detailed information about hospitalizations linked to heatwaves. Impressively, our dataset encompassed a substantial (n=471,814) hospital stays spanning from June to September over the years 2009 to 2019, representing the pre-COVID-19 period. Importantly, this dataset was exclusively derived from the Paris department.
For each hospital stay, we compiled critical data, which included the purpose of hospitalization, the residential location, and hospitalization dates. To categorize heatwave-related hospitalization reasons, we employed distinct root codes from homogeneous groups of patients (GHM) or stays (GHS), as well as International Classification of Diseases (ICD-10) codes associated with specific disease groupings.
Building upon prior research, including notable works such as Bobb’s 2014 study (7) in a similar domain, we identified 10 distinct clusters of hospitalization reasons: electrolyte disorders (excluding hypoglycemia), renal failure, urinary infections and urolithiasis, septicemia/sepsis/bacteremia, heatstroke, neuro-cardiovascular diseases, chronic heart failure, chronic respiratory diseases, diabetes mellitus, and addictions and mental health.
Through an extraction process from PMSI tables, we quantified the scale of heatwave-related hospitalizations within each department, forming a foundation for our analytical endeavors. Subsequently, we examined specific districts within Paris, supplementing our data with information from the most recent year available from INSEE’s open data and the National Building Database (BDNB). This encompassed diverse factors such as Index of Social Disadvantage (FDEP—L’indice de désavantage social) values, building ages, the percentage of residents above 75 years old, prevalence of air conditioning, and availability of elevators. These variables were carefully selected based on their potential relevance to our study. Elevators and building age, for example, were included due to their likely impact on residents’ mobility and facility safety, which could influence hospitalizations during heatwaves. Likewise, other variables were chosen based on their possible implications for the population’s vulnerability to heat effects. These choices were guided by the objective of our study to explore the complex impacts of heatwaves on hospitalizations in Paris.
Climate data
We collected climatic data from Météo France, encompassing maximum, minimum, and average temperatures recorded in each department in France. These data were gathered every 24 hours over a span of 22 years.
Our research primarily concentrates on the summer months from June to September. This specific period is chosen as it typically marks the peak of temperature highs in France, making it an essential time frame for exploring the phenomenon of heatwaves and their implications.
To substantiate our choice of these months for the study, we refer to the accompanying graph (Figure 1). This graph (Figure 1) is derived from daily data collected by Météo France over a decade, from 2009 to 2019. It distinctly portrays the monthly average temperature variations in Paris. This illustration is comprised of three separate curves that represent the average minimum (TN curve), average maximum (TX curve), and the overall average (TM curve) temperatures for each month. This graphical representation clearly demonstrates the seasonal fluctuations, characterized by colder temperatures in the winter and warmer temperatures in the summer, typical of a temperate climate like that of Paris.
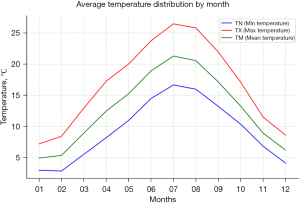
Crucially, the data reveals that the average maximum temperatures (TX curve) during these selected months are consistently higher than those in other months, often escalating to heatwave conditions. This elevation in temperature is a critical factor for our study as it directly impacts public health, making our chosen period particularly relevant for an analysis centered on health risks associated with heatwaves. Therefore, the selection of June to September is not just a reflection of the regional climatic tendencies but is also strategically aligned with our objective to assess the effects of high temperatures on health.
By focusing on this specific timeframe, our study aims to offer insightful observations and conclusions regarding the impact of extreme heat, a topic of increasing importance in the context of global climatic changes and public health planning.To determine heatwave days, we opted for a percentile-based approach. Specifically, a day was classified as a heatwave day when the maximum temperature exceeded the 90th percentile of the daily temperature distribution in the Paris department which represents 30.0 °C. This method enabled us to identify days when temperatures were exceptionally high compared to the normal range. It is important to note that the 90th percentile was calculated based on the entire dataset and not on an annual basis. We did not adjust the percentile values annually, as the climate data showed slow variability over the study period, and traditionally, fixed periods are chosen to define heatwaves, with increments occurring every 10 years. This approach indicates that the variability in temperature percentiles is gradual and supports our approximation.
To enhance organization and facilitate data analysis, we grouped heatwave days into 15-day periods. Each 15-day heatwave period was associated with one or more heatwave days, while periods without heatwaves had no such days. This approach provided us with the opportunity to examine longer-term trends and quantify the duration and intensity of heatwaves.
We chose to group heatwave days into 15-day periods to enhance the organization of our data and facilitate comprehensive data analysis. Each 15-day heatwave period was associated with one or more heatwave days, while periods without heatwaves had no such days. The decision regarding how to start the periods was based on the occurrence of heatwave events. Specifically, if a heatwave event occurred on June 15th, the corresponding 15-day heatwave period would extend from June 1st to June 15th. This approach allowed us to capture the entire duration of each heatwave event, starting from the first day temperatures exceeded the 90th percentile.
The choice of a 15-day period was made to strike a balance between capturing longer-term trends and providing granularity in our analysis. While the 4 months could be divided into eight 15-day periods, the actual division depended on the occurrence of heatwave events during that time frame. This approach allowed us to examine both the frequency and intensity of heatwaves while ensuring that we captured their full duration, which is crucial for understanding their impact on hospitalizations
Statistical analyses
A retrospective observational study was conducted to determine the impact of heatwaves on hospitalizations in the population of the Paris department from 2009 to 2019. The PMSI was used as a comprehensive data source to ensure data reliability.
For each district and fortnight, we calculated (I) the number of hospital admissions within each disease group by summing up the admissions count for each of the listed GHM codes as the primary diagnosis and aggregating these counts across GHM codes within each disease group, and (II) the hospital admission rate within each disease group for each district, taking into account the population of each district.
We employed mixed-effects log-linear regression models on multisite time-series data for hospital admissions. These models were applied separately for each disease group to investigate the correlation between the pathology rate (dependent variable) and multiple factors (independent variables). The included independent variables encompassed the count of heatwave days, maximum temperature (TX curve), the proportion of individuals aged over 75 years, the percentage of air conditioning and elevator usage, FDEP15, and the percentage of buildings aged over 75 years. Moreover, the categorical variables of year, month, and quinzaine were introduced to account for temporal effects.
Additionally, we included the percentage of buildings aged over 75 years was included to account for the age of infrastructure, as older buildings may have different heat-related vulnerabilities.
Furthermore, we introduced categorical variables for year, month, and quinzaine (a 15-day period) to account for temporal effects and seasonality, as these factors can influence both heatwave occurrence and hospitalization rates. The choice of these variables was guided by our aim to comprehensively explore the complex relationships between heatwaves, environmental variables, and demographic factors in Paris.
Risk estimates with a P value <0.05 were considered statistically significant. In our analysis, correlation coefficients are utilized to determine the strength and direction of the association between variables. A positive correlation coefficient indicates that as one variable increases, the other variable tends to increase as well. Conversely, a negative correlation coefficient suggests that as one variable increases, the other variable tends to decrease. This methodological approach allows for a nuanced understanding of the relationships between different variables under study. To facilitate interpretation, graphical representations of these correlations may be provided, assisting in the contextual understanding of the data as presented in the results section (Figure 2).
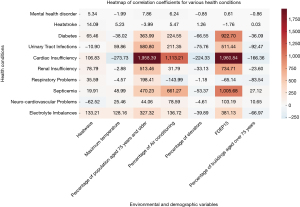
We hypothesized that the relative risk (RR) associated with heatwaves would increase with greater intensity and duration of heatwaves. Statistical analyses were conducted using R software, version 3.0.2. (http://www.r-project.org/).
Ethical Consideration
The study was conducted in accordance with the Declaration of Helsinki (as revised in 2013). The study was approved by the Montpellier University Hospital IRB (IRB Accreditation number: 198711; Approval Number: IRB-MTP_2022_03_202201074). Since the database is anonymous, obtaining consent to participate is not applicable. Access to the national hospital database (PMSI) was provided by the SNDS.
Results
During the period 2009–2019, the Paris region experienced significant fluctuations in terms of heatwaves, suggesting potential implications of climate change. A detailed analysis of these ten years reveals significant trends in the frequency and intensity of heatwave episodes.
Throughout this decade, Paris recorded a total of 139 days characterized as heatwave days, demonstrating marked interannual variability in the occurrence of these phenomena. The years 2015 and 2018 stood out with 20 and 23 heatwave days respectively, highlighting periods of extreme heat. In contrast, the year 2014 had a significantly lower frequency, with only 5 heatwave days.
Thermal analysis shows that the average maximum temperature reached its highest level in 2019, rising to 33.06 °C. More remarkably, the absolute maximum temperature was recorded in the same year, reaching 42.6 °C. This upward trend, both for average maximum temperatures and thermal peaks, indicates an intensification of heat conditions.
Temporally, the second half of July was identified as the period most impacted by heatwaves, with a total of 33 days over the decade.
Our final study population consisted of (n=2,184,193) residents living in the 20 districts (Tables 1,2).
Table 1
Index | Paris 1st arrondissement | Paris 2nd arrondissement | Paris 3rd arrondissement | Paris 4th arrondissement | Paris 5th arrondissement | Paris 6th arrondissement | Paris 7th arrondissement | Paris 8th arrondissement | Paris 9th arrondissement | Paris 10th arrondissement |
---|---|---|---|---|---|---|---|---|---|---|
Population size | 16,055 | 21,735 | 34,306 | 29,390 | 58,897 | 41,171 | 49,681 | 36,250 | 60,563 | 86,863 |
Percentage of population aged 75 years and older | 8.13% | 4.27% | 6.26% | 7.81% | 9.96% | 12.01% | 11.45% | 7.87% | 5.66% | 5.03% |
Percentage of air conditioning | 2.88% | 3.52% | 1.64% | 1.48% | 1.08% | 2.22% | 2.86% | 4.69% | 1.38% | 0.84% |
Percentage of elevators | 56.64% | 43.34% | 46.72% | 47.87% | 62.59% | 63.01% | 67.78% | 73.73% | 53.8% | 52.06% |
FDEP15 index | −4.13 | −3.9 | −4.25 | −4.24 | −4.64 | −5.46 | −5.92 | −5.62 | −4.54 | −2.78 |
Percentage of buildings aged over 75 years | 84.99% | 81.0% | 84.85% | 85.42% | 88.48% | 83.23% | 85.67% | 85.87% | 88.68% | 86.55% |
Mental health disorder | 0% | 0% | 0% | 0% | 0% | 0% | 0% | 0% | 0% | 0% |
Heatstroke | 0% | 0% | 0% | 0% | 0% | 0% | 0% | 0% | 0% | 0% |
Diabetes | 2% | 3% | 3% | 3% | 4% | 2% | 1% | 2% | 3% | 3% |
Urinary tract infections | 2% | 3% | 4% | 4% | 6% | 4% | 4% | 3% | 4% | 4% |
Cardiac insufficiency | 6% | 7% | 9% | 9% | 12% | 7% | 7% | 6% | 6% | 8% |
Renal insufficiency | 4% | 4% | 6% | 5% | 7% | 5% | 5% | 3% | 5% | 5% |
Respiratory problems | 1% | 1% | 1% | 2% | 2% | 3% | 2% | 1% | 1% | 1% |
Septicemia | 3% | 4% | 4% | 4% | 4% | 3% | 3% | 4% | 4% | 3% |
Neurocardiovascular problems | 0% | 0% | 0% | 0% | 0% | 0% | 0% | 0% | 0% | 0% |
Electrolyte imbalances | 3% | 2% | 4% | 3% | 3% | 2% | 3% | 1% | 2% | 3% |
This table provides a detailed analysis of the first 10 arrondissements of Paris, focusing on key variables. ‘Population size’ offers basic demographic context. ‘Percentage of population aged 75 years and older’ is critical for understanding vulnerability to heatwave effects, as older individuals are more likely to be affected by high temperatures. ‘Percentage of air conditioning’ and ‘Percentage of elevators’ indicate urban infrastructure that can modulate health impacts of heatwaves. The ‘FDEP15 index’, measuring social disadvantage, is included to examine how socioeconomic factors might influence resilience to heatwaves. ‘Percentage of buildings aged over 75 years’ is relevant, as older buildings may provide less effective insulation against heat. Finally, data on various health conditions such as mental health disorders, heatstroke, diabetes, urinary tract infections, cardiac insufficiency, and others, are included to directly assess the impact of heatwaves and sociodemographic variables on the health of residents in each arrondissement.
Table 2
Index | Paris 11th arrondissement | Paris 12th arrondissement | Paris 13th arrondissement | Paris 14th arrondissement | Paris 15th arrondissement | Paris 16th arrondissement | Paris 17th arrondissement | Paris 18th arrondissement | Paris 19th arrondissement | Paris 20th arrondissement |
---|---|---|---|---|---|---|---|---|---|---|
Population size | 146,028 | 140,237 | 181,233 | 136,568 | 232,668 | 167,777 | 167,975 | 193,288 | 185,571 | 195,918 |
Percentage of population aged 75 years and older |
6.7% | 8.66% | 7.9% | 9.19% | 9.18% | 11.51% | 7.35% | 5.7% | 6.33% | 6.56% |
Percentage of air conditioning | 0.43% | 0.79% | 0.61% | 1.09% | 0.77% | 2.37% | 0.91% | 0.52% | 0.83% | 0.42% |
Percentage of elevators | 57.47% | 71.75% | 79.54% | 72.59% | 78.35% | 80.61% | 66.17% | 57.61% | 76.15% | 69.8% |
FDEP15 index | −3.09 | −3.1 | −2.18 | −3.19 | −3.93 | −5.15 | −3.64 | −1.66 | −0.81 | −1.33 |
Percentage of buildings aged over 75 years |
82.96% | 81.77% | 76.26% | 80.79% | 77.38% | 80.94% | 88.45% | 85.16% | 76.77% | 74.12% |
Mental health disorder | 0% | 0% | 0% | 0% | 0% | 0% | 0% | 0% | 0% | 0% |
Heatstroke | 0% | 0% | 0% | 0% | 0% | 0% | 0% | 0% | 0% | 0% |
Diabetes | 5% | 4% | 3% | 1% | 2% | 2% | 1% | 1% | 1% | 2% |
Urinary tract infections | 3% | 5% | 3% | 3% | 4% | 4% | 3% | 5% | 3% | 3% |
Cardiac insufficiency | 8% | 9% | 4% | 6% | 9% | 11% | 9% | 9% | 6% | 4% |
Renal insufficiency | 9% | 6% | 3% | 7% | 3% | 5% | 7% | 6% | 3% | 4% |
Respiratory problems | 1% | 2% | 1% | 0% | 3% | 1% | 3% | 1% | 1% | 0% |
Septicemia | 9% | 3% | 3% | 3% | 3% | 4% | 3% | 5% | 4% | 4% |
Neurocardiovascular problems | 0% | 0% | 0% | 0% | 1% | 0% | 0% | 0% | 0% | 0% |
Electrolyte imbalances | 4% | 3% | 0% | 3% | 2% | 3% | 4% | 3% | 3% | 3% |
This table offers a comprehensive view of the population size, age demographics, infrastructure, social disadvantage, and health outcomes in these arrondissements. The ‘Population size’ provides a baseline for understanding the scope of each arrondissement. The ‘Percentage of population aged 75 years and older’ is a key indicator for assessing vulnerability to heatwave-related health issues, as older populations are often more susceptible. ‘Percentage of air conditioning’ and ‘Percentage of elevators’ offer insights into the infrastructure that can affect the residents’ ability to cope with heatwaves. The ‘FDEP15 index’ is included to examine the relationship between social disadvantage and health outcomes during heatwaves. The ‘Percentage of buildings aged over 75 years’ indicates the prevalence of older buildings, which may be less equipped to handle extreme temperatures. Health-related data, including prevalence of mental health disorders, heatstroke, diabetes, urinary tract infections, cardiac insufficiency, renal insufficiency, respiratory problems, septicemia, neurocardiovascular problems, and electrolyte imbalances, are crucial for understanding the direct impact of heatwaves and sociodemographic factors on public health in these specific areas of Paris.
Demographic and structural characteristics across Parisian districts
Tables 1,2 elucidate the demographic distribution and pertinent architectural attributes across Parisian districts. These districts manifest significant variability in parameters such as population demographics, the proportion of residents aged ≥75 years, air conditioning prevalence, elevator accessibility, the FDEP15 index, and the percentage of edifices surpassing 75 years of age.
For illustrative purposes, the Paris 5th district is characterized by a resident count of 58,897, of which 9.96% are aged 75 years or older. The prevalence of air conditioning in residential units is 1.08%. Additionally, 62.59% of the district’s structures are equipped with elevators, and 88.48% of its buildings have been standing for over 75 years.
Tables 1,2 show variations in medical conditions across different districts. Conditions such as mental health disorders and heatstroke were not present. The prevalence of diabetes varies, with 5% in the Paris 11th district and 4% in the Paris 5th district. Urinary tract infections occur at a rate of 6% in districts like the Paris 5th, and cardiac insufficiency reaches a peak of 12% in the same district.
Risk of hospitalization during fortnights with heatwaves compared to fortnights without heatwaves
Our analysis has identified notable relationships between various factors and health outcomes (Table 3).
Table 3
Hospitalizations clusters | Heatwave | Maximum temperature | % of population aged 75 years and older | % of air conditioning | % of elevators | FDEP15 index | % of buildings aged over 75 years |
---|---|---|---|---|---|---|---|
Mental health disorder | 5.34 (0.1181) | −1.99 (0.2852) | 7.86 (0.0521) | 6.24 (0.3015) | −0.85 (0.1542) | 0.61 (0.939) | −0.86 (0.5763) |
Heatstroke | 14.09 (0.0003) | 5.23 (0.0138) | −3.99 (0.3874) | 5.47 (0.4272) | 1.26 (0.0666) | −1.76 (0.847) | 0.03 (0.9883) |
Diabetes | 65.46 (0.4392) | −38.02 (0.4095) | 363.99 (0.0003) | 224.55 (0.1338) | −66.55 (0.0) | 922.7 (0.0) | −36.09 (0.343) |
Urinary tract infections | −10.9 (0.8984) | 59.86 (0.1979) | 580.8 (0.0) | 211.35 (0.1617) | −75.76 (0.0) | 511.44 (0.011) | −92.47 (0.0161) |
Cardiac insufficiency | 106.83 (0.501) | −273.73 (0.0016) | 1,958.39 (0.0) | 1,113.21 (0.0001) | −224.33 (0.0) | 1,960.84 (0.0) | −166.36 (0.0199) |
Renal insufficiency | 78.79 (0.3444) | −2.88 (0.9494) | 513.46 (0.0) | 31.79 (0.8293) | −33.13 (0.0237) | 734.71 (0.0) | −23.6 (0.5289) |
Respiratory problems | 35.59 (0.6007) | −4.57 (0.9017) | 198.41 (0.0139) | −143.99 (0.2314) | −1.18 (0.9215) | −65.14 (0.684) | −83.54 (0.0063) |
Septicemia | 19.91 (0.83) | 48.99 (0.3321) | 470.23 (0.0) | 661.27 (0.0001) | −53.37 (0.0011) | 1,005.6 (0.0) | 27.12 (0.5154) |
Neurocardiovascular problems | −62.52 (0.0314) | 25.46 (0.1076) | 44.06 (0.2008) | 78.59 (0.1263) | −4.61 (0.3667) | 103.19 (0.131) | 10.65 (0.4149) |
Electrolyte imbalances | 133.21 (0.0637) | 128.16 (0.0011) | 327.32 (0.0001) | 136.72 (0.282) | −39.89 (0.0016) | 381.13 (0.024) | −66.97 (0.0382) |
This table presents the results of a multiple regression analysis conducted to assess the impact of various factors on health outcomes in the Parisian districts. The dependent variables include health-related outcomes, such as ‘Mental health disorder’, ‘Heatstroke’, ‘Diabetes’, ‘Urinary tract infections’, ‘Cardiac insufficiency’, ‘Renal insufficiency’, ‘Respiratory problems’, ‘Septicemia’, ‘Neurocardiovascular problems’, and ‘Electrolyte imbalances’. The independent variables considered in the analysis are ‘Heatwave’, ‘Maximum temperature’, ‘% of population aged 75 years and older’, ‘% of air conditioning’, ‘% of elevators’, ‘FDEP15 index’, and ‘% of buildings aged over 75 years’. The coefficients and P values are presented for each health outcome, reflecting the strength and significance of the relationships with the independent variables. The results provide insights into how heatwaves, temperature, sociodemographic characteristics, and infrastructure may influence various health conditions in the Parisian districts.
For “Addictions and Mental Health” an additional day of a heatwave corresponded to an increase of 5.34 cases (P=0.12, adjusted by a factor of 100,000). Moreover, each unit of 1 degree Celsius increase in maximum temperature (TX curve) was associated with a decrease of 1.99 cases (P=0.29). A 1% increment in the population aged over 75 years correlated with an increase of 7.86 cases (P=0.05).
In the context of “Heat Stroke”, each additional heatwave day resulted in a significant 14.09 case rise (P<0.001).
A unit increase in TX curve led to 5.23 more cases (P=0.01).
For “Diabetes”, a 1% increase in the population over 75 years was linked with 363.99 additional cases (P<0.001). The FDEP15 variable stood out with an association coefficient of 922.7 (P<0.001).
“Urinary Infections” displayed a marked association with the older population segment, with a 1% increase correlating with 580.8 additional cases (P<0.001). The FDEP15 variable also showed significance, with a coefficient of 511.44 (P=0.01).
For “Chronic Heart Failure”, a 1% increase in the older population corresponded to a 1,958.39 case increase (P<0.001). The air conditioning percentage and the FDEP15 variable had coefficients of 1,113.21 (P<0.001) and 1,960.84 (P<0.001) respectively.
In the realm of “Renal Failure”, the older population’s 1% increment was associated with 513.46 more cases (P<0.001), and the FDEP15 variable exhibited a coefficient of 734.71 (P<0.001).
For “Chronic Respiratory Diseases”, the older population’s 1% increase aligned with 198.41 additional cases (P=0.01).
“Septicemias” highlighted the influence of age and environment, with a 1% rise in the older population leading to 470.23 more cases (P<0.001). The influence of air conditioning was also significant, with a coefficient of 661.27 (P<0.001), alongside the FDEP15 variable at 1,005.68 (P<0.001).
Regarding “Neuro-cardiovascular Diseases”, each added heatwave day was inversely linked with 62.52 fewer cases (P=0.03).
An additional heatwave day was connected with 133.21 more ‘Electrolyte Disorders’ cases (P=0.06), and each TX curve unit increment resulted in 128.16 additional cases (P=0.001). A 1% rise in the older population was associated with 327.32 extra cases (P<0.001).
Discussion
Key findings
Our study, which analyzed the intersection of heatwaves, environmental variables, and demographic factors in Paris, has brought forth several pivotal findings. During the 2009–2019 period, the Paris region experienced considerable variations in heatwaves, with a total of 139 heatwave days recorded, highlighting the potential impact of climate change. The year 2019 marked a peak in temperatures, with an average maximum of 33.06 °C and an absolute record of 42.6 °C, indicating an increase in heat intensity. Late July emerged as the most affected period.
The study encompassed 2,184,193 residents from Paris’s 20 districts. Demographic and structural analyses revealed significant disparities among districts, particularly in terms of the percentage of elderly residents, prevalence of air conditioning, elevator accessibility, and the proportion of old buildings. For instance, in the 5th district, 9.96% of the population was over 75 years old, air conditioning was present in 1.08% of dwellings, and 88.48% of the buildings were over 75 years old.
Variations in medical conditions across districts were noted, with varying prevalences of diabetes, urinary tract infections, and cardiac insufficiency. The analysis also revealed significant correlations between heatwaves, demographic and environmental factors, and hospitalization rates. Marked increases in cases were linked to additional heatwave days, temperature increases, and a higher proportion of the elderly population, especially for conditions such as heat stroke, diabetes, urinary infections, and chronic heart failure. The intricate interplay of these factors underscores the profound impact of heatwaves on public health in dense urban areas like Paris.
Strengths and limitations
One of the primary strengths of our study lies in the comprehensive data sourcing from the SNDS and the ATIH. The extensive dataset, encompassing over 471,814 hospital stays spanning a decade, offers a robust foundation for our conclusions. The meticulous categorization of hospitalizations, coupled with the use of mixed-effects log-linear regression models, further bolsters the reliability of our findings.
However, our study also has its limitations. Firstly, the retrospective nature of our methodology, while comprehensive, may not capture all potential confounding variables. This could introduce biases or overlook certain nuances in the data. Secondly, our study predominantly focused on Paris, implying that the findings might not be directly transferable to other urban areas with distinct demographic and environmental characteristics. It is aldo possible that due to the extreme heat, patients may have stayed home when experiencing illness.
An additional limitation might be that the study focuses on a 4-month block, broken into 15-day blocks, as opposed to the greater granularity that might be achieved via weekly or even daily groupings.
Another significant limitation is the inclusion of planned hospitalizations in our data extraction. Planned hospitalizations refer to planned admissions, which are not emergent or based on immediate medical necessity. Including these in our dataset might skew the results, as they are not directly influenced by heatwaves or other immediate environmental factors. This inclusion could potentially inflate the number of hospitalizations attributed to heatwave.
Comparison with similar research
The implications of heatwaves on human health, especially against the backdrop of a changing global climate, have been extensively explored. Our findings, particularly regarding the elderly population’s vulnerability to heatwaves, align with those from other research. For instance, a study conducted in Adelaide, Australia by Zhang et al., highlighted a significant risk of heat-related morbidity among the elderly, with heart disease and kidney disease emerging as notable risk factors (11). This observation underscores the intricate relationship between preexisting health conditions and heat-related vulnerabilities.
Protective measures identified by Zhang et al., such as the presence of air-conditioning and increased social activities, are consistent with findings from Van den Wyngaert et al., which underscored the detrimental effects of social isolation during heatwaves (12). Such parallels accentuate the universal nature of these protective factors, suggesting their potential applicability across various settings, including Paris.
Adélaïde et al. highlighted the significant economic burden heatwaves place on the French health system (13). The majority of these impacts were attributed to premature mortality, particularly in the elderly demographic. This aligns with our observation of the pronounced vulnerability of the elderly in Paris. Interestingly, while our study emphasized the hospitalization rates during heatwaves, Adélaïde et al. revealed that morbidity represented only a small fraction of the economic burden. This suggests that while hospitalizations may surge, the economic implications of these hospitalizations are overshadowed by the broader societal and economic impacts of premature deaths.
In addition to our findings, it is pertinent to consider the similarities and differences with the results reported by Adélaïde et al. (13). While our research highlights the role of environmental indicators during the 2019 heatwave in France, the study by Adélaïde et al. emphasizes the significant economic consequences, particularly due to the very high diurnal temperatures. This concurrence underscores the complex implications of extreme heat events.
Furthermore, our analysis sheds light on the temporal nature of heatwaves in Paris, comparing data from 2009 and 2019 (Figure 3). We observed an increase in the frequency of heatwave days, rising from 12 days in 2009 to 18 days in 2019. This uptick suggests a trend of increasing heatwave frequency over the studied period. This observation, in correlation with concerns related to climate change, indicates a potential exacerbation of extreme summer temperatures. Thus, our study, complementing that of Adélaïde et al., highlights the necessity for more robust adaptation strategies in response to these increasingly frequent phenomena.
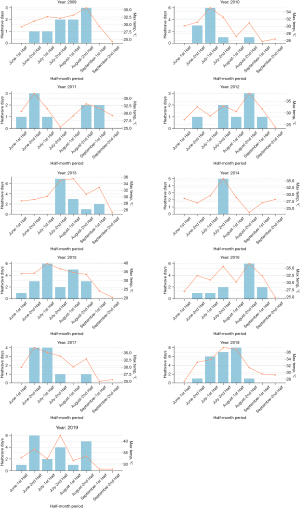
Another study by Van den Wyngaert et al. focused on the elderly population in nursing homes (12). Their findings resonate with our observations on the heightened vulnerability of the elderly in Paris. While we identified a significant determinant in hospitalization rates for those aged over 75 years during heatwaves, Van den Wyngaert et al. found a direct association of heatwaves with mortality in nursing homes but not with hospitalizations (12). This discrepancy between mortality and morbidity in the elderly, especially in nursing homes, suggests that while heatwaves may lead to an immediate health crisis, the long-term implications, such as death, are more pronounced in this demographic.
In the other hand the study by Bobb et al. from the Department of Biostatistics (7), offers a comprehensive insight into the effects of heatwaves on hospital admissions among older adults in the United States (7).
Bobb et al. identified a significant association between heat exposure and elevated hospital admissions for fluid and electrolyte disorders, heat stroke, septicemia, urinary tract infection, and renal failure in a vast population of older adults. Their findings indicated that the risks were more pronounced during more extended and more extreme heatwave periods and were most significant on the heatwave day, remaining elevated for 1 to 5 subsequent days (7). This observation aligns with our study, emphasizing the immediate and lingering effects of heatwaves on health.
Interestingly, while our study highlighted the hospitalization rates during heatwaves in Paris, Bobb et al. provided a broader perspective by examining 214 diseases classified from 15,072 ICD-9 codes in a population of 23.7 million older adults per year for 12 years from 1,943 urban and rural counties (7). Their approach, which considered a broad range of possible outcomes without an a priori hypothesis, offers a comprehensive understanding of the physiological events caused by exposure to extreme heat.
In conclusion, our study offers a comprehensive insight into the effects of heatwaves on hospitalization rates in Paris, particularly among the elderly. However, when juxtaposed with similar research, it becomes evident that the broader implications of heatwaves—whether economic or health-related—are profound and multifaceted. The consistent emphasis across studies on the vulnerability of the elderly further underscores the need for targeted interventions and policies to protect this demographic.
Interpretation of findings
Our study’s findings offer a comprehensive perspective on the influence of heatwaves on hospitalizations within the Paris department. A consistent theme emerging from the data is the pronounced vulnerability of the elderly population to heatwaves. The age demographic, especially the proportion of individuals aged over 75 years, was a significant predictor for a multitude of health conditions. This aligns with global literature that has consistently highlighted the heightened susceptibility of the elderly to extreme temperature events due to their diminished physiological ability to respond to heat stress.
Environmental factors and building infrastructure also played a pivotal role. The FDEP15 variable, which likely represents an environmental or infrastructural factor, was a recurrent predictor across several conditions. Its significance in conditions such as “Diabetes”, “Urinary Infections”, “Chronic Heart Failure”, “Renal Failure”, and “Septicemias” implies that specific environmental or structural attributes of Parisian districts are integral to heatwave-related hospitalizations. Furthermore, the prevalence of air conditioning in districts was notably associated with conditions like “Chronic Heart Failure” and “Septicemias”. This observation underscores the potential protective role of air conditioning during heatwaves, a finding with profound implications for urban planning and public health interventions.
The direct repercussions of heat and heatwaves were evident in conditions intrinsically linked to heat stress. For instance, an additional heatwave day led to a marked rise in “Heat Stroke” cases. Similarly, conditions like “Electrolytic Disorders”, which can be exacerbated by dehydration during heatwaves, saw an uptick with each added heatwave day and TX curve unit increment. However, the relationship between heat and certain conditions, such as “Neuro-cardiovascular Diseases”, was more complex, as evidenced by the inverse relationship with added heatwave days.
The variability in demographic and architectural attributes across Parisian districts was also striking. For example, the Paris 5th district had a pronounced prevalence of conditions like urinary tract infections and cardiac insufficiency compared to other districts. Such district-specific insights can be instrumental in guiding targeted interventions and resource allocation during heatwave events.
It is particularly remarkable that, for conditions related to mental health and heat strokes, the data shows different trends compared to other health conditions. For example, for “Addictions and Mental Health”, we observed that an additional day of a heatwave corresponded to an increase of 5.34 cases, although this relationship was not statistically significant at a conventional level. However, the increase in the population aged over 75 years was significantly correlated with an increase of 7.86 cases of this condition. These findings suggest that demographic factors may play a key role in how heatwaves affect the mental health of the population.
As for “Heat Stroke”, it is clear that this condition reacts much more sensitively to heatwaves. Each additional day of a heatwave results in a significant increase of 14.09 cases, while an increase of one unit in the maximum temperature (TX curve) is associated with an additional 5.23 cases. These results are consistent with what one would expect, as heatstroke is directly linked to prolonged exposure to high temperatures.
Lastly, while many factors were associated with increased hospitalizations, some appeared to have potential protective effects. For instance, an increase in maximum temperature (TX curve) correlated with a decrease in “Addictions and Health” cases. This inverse relationship suggests that certain conditions might experience reduced exacerbations during heatwaves, though the underlying mechanisms merit further exploration. Additionally, the review provided an interesting suggestion that heatwaves may encourage patients to stay home during exacerbations, which presents an equally plausible explanation for the observed decrease in mental health and addiction exacerbations during periods of high heat.
In summary, our study paints a multifaceted picture of the challenges posed by heatwaves on the health of Parisians. The insights gleaned are invaluable for a range of stakeholders, from policymakers and healthcare providers to urban planners, as they strategize to counteract the adverse effects of future heatwaves.
Implications and actions needed
The findings from our study have profound implications for public health policies and urban planning in Paris. Given the heightened vulnerability of the elderly, there is a pressing need for targeted interventions. This could include community outreach programs, ensuring the elderly have access to air-conditioned spaces, and public health campaigns educating about the risks of heatwaves.
Furthermore, urban planners and policymakers should consider incorporating more green spaces and water bodies in urban areas, which can act as natural coolants. The healthcare infrastructure should also be bolstered to handle the increased hospitalizations during heatwave periods.
Generalizability
While our study provides valuable insights into the effects of heatwaves on hospitalization rates in Paris, caution should be exercised when generalizing these findings to other regions. Paris, with its unique demographic and environmental characteristics, might respond differently to heatwaves compared to other urban areas. However, the methodology and the analytical framework used in this study can serve as a blueprint for similar research in other urban centers, helping to understand the global implications of heatwaves in the era of climate change.
Conclusions
Amid the pressing challenges posed by climate change, heatwaves emerge as a phenomenon with tangible and concerning impacts on public health in Paris. Our findings not only validate this broad concern but also shed light on significant nuances regarding the most vulnerable demographic groups, notably the elderly. These nuances offer critical insights for policymakers and healthcare professionals tasked with addressing the health impacts of climate change.
It is evident that environmental and demographic variables play a pivotal role in the repercussions of heatwaves on hospitalization rates. This revelation underscores the need for an integrated and nuanced approach to public health in the face of climatic challenges. As we have demonstrated, the distinct characteristics of urban zones like Paris further accentuate the urgency to adopt strategies tailored to this unique context.
While recognizing the immediate risks, our focus must also extend to the long-term implications of these climatic events. Future research should strive to understand both the direct impacts and the potential ripple effects of heatwaves, thereby offering a comprehensive view of the looming health challenges. This holistic understanding is essential for crafting sustainable and effective public health policies.
In light of these discoveries, a close collaboration between policymakers, healthcare professionals, and researchers is paramount. Together, they can forge proactive and effective strategies, ensuring the safety and well-being of residents in an ever-evolving environment. By working together, we can develop and implement measures that not only mitigate the immediate health risks posed by heatwaves but also contribute to the long-term resilience of our communities in the face of climate change.
Acknowledgments
Funding: This study was supported by
Footnote
Provenance and Peer Review: This article was commissioned by the Guest Editors (Michael K. Gusmano & Victor G. Rodwin) for the series “Health Systems and Health in World Cities: Challenges for the Future” published in Journal of Hospital Management and Health Policy. The article has undergone external peer review.
Reporting Checklist: The authors have completed the STROBE reporting checklist. Available at https://jhmhp.amegroups.com/article/view/10.21037/jhmhp-23-115/rc
Peer Review File: Available at https://jhmhp.amegroups.com/article/view/10.21037/jhmhp-23-115/prf
Conflicts of Interest: All authors have completed the ICMJE uniform disclosure form (available at https://jhmhp.amegroups.com/article/view/10.21037/jhmhp-23-115/coif). The series “Health Systems and Health in World Cities: Challenges for the Future” was commissioned by the editorial office without any funding or sponsorship. The authors have no other conflicts of interest to declare.
Ethical Statement: The authors are accountable for all aspects of the work in ensuring that questions related to the accuracy or integrity of any part of the work are appropriately investigated and resolved. The study was conducted in accordance with the Declaration of Helsinki (as revised in 2013). The study was approved by the Montpellier University Hospital IRB (IRB Accreditation number: 198711; Approval Number: IRB-MTP_2022_03_202201074). Since the database is anonymous, obtaining consent to participate is not applicable. Access to the national hospital database (PMSI) was provided by the SNDS.
Open Access Statement: This is an Open Access article distributed in accordance with the Creative Commons Attribution-NonCommercial-NoDerivs 4.0 International License (CC BY-NC-ND 4.0), which permits the non-commercial replication and distribution of the article with the strict proviso that no changes or edits are made and the original work is properly cited (including links to both the formal publication through the relevant DOI and the license). See: https://creativecommons.org/licenses/by-nc-nd/4.0/.
References
- Intergovernmental Panel on Climate Change. Climate Change 2014: Impacts, Adaptation, and Vulnerability. Part A: Global and Sectoral Aspects. Cambridge, UK: Cambridge University Press, 2014.
- Smith KR, Woodward A, Campbell-Lendrum D, et al. Human health: impacts, adaptation, and cobenefits. In: Climate Change 2014: Impacts, Adaptation, and Vulnerability. Part A: Global and Sectoral Aspects. Cambridge, UK: Cambridge University Press, 2014:709-54.
- Fouillet A, Rey G, Wagner V, et al. Has the impact of heat waves on mortality changed in France since the European heat wave of summer 2003? A study of the 2006 heat wave. Int J Epidemiol 2008;37:309-17. [Crossref] [PubMed]
- Adélaïde L, Chanel O, Pascal M. Évaluation monétaire des effets sanitaires des canicules en France métropolitaine entre 2015 et 2020. Bulletin Epidemiologique Hebdomadaire 2021;12:215-23.
- Campbell S, Remenyi TA, White CJ, et al. Heatwave and health impact research: A global review. Health Place 2018;53:210-8. [Crossref] [PubMed]
- Yin P, Chen R, Wang L, et al. The added effects of heatwaves on cause-specific mortality: A nationwide analysis in 272 Chinese cities. Environ Int 2018;121:898-905. [Crossref] [PubMed]
- Bobb JF, Obermeyer Z, Wang Y, et al. Cause-specific risk of hospital admission related to extreme heat in older adults. JAMA 2014;312:2659-67. [Crossref] [PubMed]
- Institut national de la statistique et des études économiques (INSEE). Rapport sur les épisodes de chaleur de 2019 en France. February 2020. Available online: https://www.insee.fr/fr/statistiques/4299803
- Martínez-Solanas È, Quijal-Zamorano M, Achebak H, et al. Projections of temperature-attributable mortality in Europe: a time series analysis of 147 contiguous regions in 16 countries. Lancet Planet Health 2021;5:e446-54. [Crossref] [PubMed]
- Song X, Wang S, Hu Y, et al. Impact of ambient temperature on morbidity and mortality: An overview of reviews. Sci Total Environ 2017;586:241-54. [Crossref] [PubMed]
- Zhang Y, Nitschke M, Krackowizer A, et al. Risk factors of direct heat-related hospital admissions during the 2009 heatwave in Adelaide, Australia: a matched case-control study. BMJ Open 2016;6:e010666. [Crossref] [PubMed]
- Van den Wyngaert I, De Troeyer K, Vaes B, et al. Impact of Heat Waves on Hospitalisation and Mortality in Nursing Homes: A Case-Crossover Study. Int J Environ Res Public Health 2021;18:10697. [Crossref] [PubMed]
- Adélaïde L, Chanel O, Pascal M. Health effects from heat waves in France: an economic evaluation. Eur J Health Econ 2022;23:119-31. [Crossref] [PubMed]
Cite this article as: Sabri R, Ahikki M, Baehr C, Schneider M, Mercier G. Impact of heatwaves, demographics, and environment on hospitalization rates in Paris. J Hosp Manag Health Policy 2024;8:3.