High hospital volume is associated with more consistent long-term mortality rates
Introduction
Long-term survival following cancer treatment is a widely accepted metric used to evaluate the quality of cancer care and varies between hospitals (1-3). Long-term survival takes years to evaluate, and these metrics reflect care quality from many years prior. Long-term survival has unknown applicability as a quality measure to evaluate current performance. It is important to determine if the structural lag in measurement limits the value of long-term survival measures meaningless as a tool for assessing current hospital performance. The study assesses the stability of hospitals’ performance over time based on its cancer patients’ four-year survival. We hypothesized that hospitals’ four-year mortality ratio would be consistent over time, implying that patients could use such information when deciding where to get care. Additionally, since decades of research have demonstrated a relationship between higher surgical volume and better outcomes for hospitals, we set out to explore consistency by hospital volume (1,4).
Methods
Data sources
Medicare Fee-for-Service (FFS) 100% Research Identifiable Files were used. We assigned each patient to a single hospital using claims for cancer-related healthcare encounters (i.e., the same eligible claims used to assign index date).
Study sample
Patients treated with chemotherapy, radiotherapy, or surgery at an acute care or critical access hospital in 2006, 2011, 2012, and 2013 (index years) were in the analysis and we obtained 4-year mortality ratios for each hospital in 2010, 2015, 2016, and 2017 respectively (data available from our previous studies (3-5). Hospitals were identified across index years via the Centers for Medicare and Medicaid Services Certification Numbers. Hospitals were excluded if they could not be matched across years or had fewer than 50 Medicare FFS patients with cancer treatment in any index year or were a psychiatric, rehabilitation, children’s psychiatric, acute long-term care hospital, alcoholism and other chemical dependency, and children’s acute long-term care hospital.
Hospitals were then divided into quartiles based on their total FFS Medicare patient volume combined across index years. As per prior work, Medicare FFS beneficiaries were included if they initiated cancer treatment or management of recurrent disease in the index year indicated by an absence of claims for cancer in the previous year (3-6).
Statistical analyses
For each hospital in each index year, a four-year risk-adjusted mortality ratio was calculated. Ratios were determined by dividing the observed number of deaths by an expected number of deaths, using previously described methods (3) In brief, the 3M Clinical Risk Group (CRG) risk adjustment model (7) in combination with adjustments for age and median income level of the zip code of residence served to adjust for differences in patient severity and demographics. A risk-adjusted mortality ratio below one indicates that a hospital performed better than expected, whereas a ratio greater than one means a hospital had higher mortality than what was expected.
We compared four-year risk-adjusted mortality ratios for each hospital between pairs of index years. For example, we compared hospitals’ outcomes based on index years 2006 and 2011 which reflects a five-year measurement time gap. We conducted separate analyses for each pairing of index years with different time gaps. The time gaps included index years separated by one, two, five, six, and seven years. Mortality ratios were averaged for the two comparisons with a one-year gap (2011 to 2012; and 2012 to 2013).
We calculated Pearson correlations to assess if estimates of the hospitals’ outcomes based on the two index years were related. We ran separate Pearson correlations for each pair of index years tested, which reflected the different time gaps (e.g., one-year gap, five-year gap). We also stratified these analyses by hospital volume.
The study was considered exempt research by the Memorial Sloan Kettering Cancer Center Institutional Review Board and CMS granted data use approval. R software version 3.5.1 was used for analyses.
Results
There were 1,640 hospitals and more than 350,000 FFS Medicare patients in each index year. Hospitals’ four-year risk-adjusted mortality ratios ranged from 0.60 to 1.44 in 2006.
Figure 1 shows correlation statistics for hospitals’ four-year mortality ratio in relation to the number of years elapsed between measurement period stratified by hospital volume. The highest volume hospitals (defined as 335 Medicare patients or more) have higher correlations compared to the other hospital categories. The plot also shows that the longer the elapsed time between measurement periods, the lower the correlation irrespective of hospital volume.
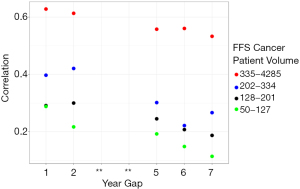
Discussion
We found that four-year risk-adjusted mortality ratios of cancer patients are reasonably consistent for larger volumes of FFS Medicare patients, but less so for hospitals with lower volumes. Among higher volume hospitals (defined as 335 Medicare patients or more), long-term mortality could be added to the list cancer care quality metrics (8,9). and be a useful metric for patients’ when selecting hospital for cancer care.
The proximity in the time of the measurement periods should have no effect on how strongly correlated outcomes were between two measurement periods if they varied by chance. It is unknown if higher volume hospitals have more consistent outcomes over time because their performance is more consistent or because the sample size required by our inclusion criteria may reduce random variability. Related research has shown that higher volume hospitals have superior survival outcomes compared to lower volume hospitals (1,2). However, the relationship between consistency and superior outcomes is not well defined, and further research is needed to assess this relationship. It would help to determine if higher volume hospitals have superior and more consistent outcomes compared to lower volume hospitals.
Consistency in longer term outcomes for lower and medium sized hospitals was less conclusive in this analysis. It is known that obtaining reliable outcome measurements for lower volume hospitals has been an issue in assessing quality measurements (10). Therefore, it is hard to determine if the lack of consistency for lower and medium sized hospitals is related to the difficulty of obtaining reliable measurements (statistical variation) or they simply have less consistent outcomes (performance variation).
Acknowledgments
Funding: This work was supported by P30 CA 008748 from the National Institutes of Health Core to Memorial Sloan Kettering Cancer Center.
Footnote
Provenance and Peer Review: This article was a standard submission to the journal. The article has undergone external peer review.
Conflicts of Interest: All authors have completed the ICMJE uniform disclosure form (available at http://dx.doi.org/10.21037/jhmhp-20-118). PBB reports personal fees from Mercer, personal fees and non-financial support from United Rheumatology, personal fees from Foundation Medicine, personal fees from Grail, personal fees from Morgan Stanley, personal fees from NYS Rheumatology Society, personal fees and non-financial support from Oppenheimer & Co, personal fees from Cello Health, personal fees, non-financial support and other from Oncology Analytics, personal fees from Anthem, personal fees from Magellan Health, personal fees and non-financial support from Kaiser Permanente Institute for Health Policy, personal fees and non-financial support from Congressional Budget Office, personal fees and non-financial support from America’s Health Insurance Plans, grants from Kaiser Permanente, grants from Arnold Ventures, personal fees and non-financial support from Geisinger, personal fees from EQRx, personal fees from Meyer Cancer Center of Weill Cornell Medicine, personal fees from National Pharmaceutical Council, outside the submitted work. The other authors have no conflicts of interest to declare.
Ethical Statement: The authors are accountable for all aspects of the work in ensuring that questions related to the accuracy or integrity of any part of the work are appropriately investigated and resolved.
Open Access Statement: This is an Open Access article distributed in accordance with the Creative Commons Attribution-NonCommercial-NoDerivs 4.0 International License (CC BY-NC-ND 4.0), which permits the non-commercial replication and distribution of the article with the strict proviso that no changes or edits are made and the original work is properly cited (including links to both the formal publication through the relevant DOI and the license). See: https://creativecommons.org/licenses/by-nc-nd/4.0/.
References
- Bach PB, Cramer LD, Schrag D, et al. The influence of hospital volume on survival after resection for lung cancer. N Engl J Med 2001;345:181-8. [Crossref] [PubMed]
- Birkmeyer JD, Sun Y, Wong SL, et al. Hospital volume and late survival after cancer surgery. Ann Surg 2007;245:777-83. [Crossref] [PubMed]
- Pfister DG, Rubin DM, Elkin EB, et al. Risk Adjusting Survival Outcomes in Hospitals That Treat Patients With Cancer Without Information on Cancer Stage. JAMA Oncol 2015;1:1303-10. [Crossref] [PubMed]
- Lavery JA, Lipitz-Snyderman A, Li DG, et al. Identifying Cancer-Directed Surgeries in Medicare Claims: A Validation Study Using SEER-Medicare Data. JCO Clin Cancer Inform 2019;3:1-24. [Crossref] [PubMed]
- Lavery JA, Lipitz-Snyderman A, Li DG, et al. Assessing whether cancer stage is needed to evaluate measures of hospital surgical performance. J Eval Clin Pract 2020;26:66-71. [Crossref] [PubMed]
- Lipitz-Snyderman A, Vater L, Curry M, et al. Cancer hospital advertising and outcomes: trust the messenger? Lancet Oncol 2019;20:760-2. [Crossref] [PubMed]
- Hughes JS, Averill RF, Jon E, et al. Clinical Risk Groups (CRGs): A Classification System for Risk-Adjusted Capitation-Based Payment and Health Care Management. Med Care 2004;42:81-90. [Crossref] [PubMed]
- Yang A, Chimonas S, Bach PB, et al. Critical Choices: What Information Do Patients Want When Selecting a Hospital for Cancer Surgery? J Oncol Pract 2018;14:e505-12. [Crossref] [PubMed]
- Chimonas S, Fortier E, Li DG, Lipitz-Snyderman A. Facts and Fears in Public Reporting: Patients' Information Needs and Priorities When Selecting a Hospital for Cancer Care. Med Decis Making 2019;39:632-41. [Crossref] [PubMed]
- Glance LG, Li Y, Dick AW. Quality of Quality Measurement: Impact of Risk Adjustment, Hospital Volume, and Hospital Performance. Anesthesiology. 2016;125:1092-102. [Crossref] [PubMed]
Cite this article as: Curry M, Lipitz-Snyderman A, Rubin DM, Li DG, Duck E, Radzyner M, Bach PB. High hospital volume is associated with more consistent long-term mortality rates. J Hosp Manag Health Policy 2021;5:42.