Technical efficiency analysis of health facilities in Haiti: a stochastic frontier approach
Introduction
Health outcomes in Haiti can be characterized as one of the poorest in the Caribbean region. In 2013 infant mortality rate was 55 per 1,000 live births and maternal mortality ratio was 380 per 100,000 live births in the country. These figures were significantly higher than the regional averages (1). The country had also one of the highest numbers of HIV incidence and prevalence. The general HIV related mortality was estimated to be 13% (2). These factors contributed to the country’s relatively short life expectancy in the region, which is estimated to be 63 years (3).
Haiti’s poor health outcomes are partly attributed to lower technical efficiency (TE) of health production in the country (3). TE is a health production process performance indicator and it measures the ratio of health outcomes produced to maximum possible health outcomes that can be produced, given health inputs (4). A recent World Bank report indicated that TE in Haiti was 4% in dispensaries, 9% in health centers without bed (HCWOB), and 30% in health centers with bed (HCWB) (3). This implies that dispensaries would have maximized health outcome by 96% given inputs or they would have reduced health inputs by 96% to produce the same health outcome.
In contrast, Moreno-Serra et al. (5) characterized Haitian health system as one of the most efficient in Latin America and the Caribbean region. They estimated the TE level of the country at 100% for most of the health outcome indicators used in their study. A precise TE measures can help benchmarking of performance in program planning, monitoring progress, and evaluating achievements (6,7). However, depending on variations in units of analysis, outcome and input indicators, and method of analysis, TE score estimates may differ from study to study (8).
The objective of this study is to estimate TE scores for health facilities in Haiti using a Stochastic Frontier Analysis (SFA) approach (9). Beyond estimating technical inefficiency, SFA allow to consider stochastic errors and facility heterogeneity in health production models (4,10). We present the following article in accordance with the MDAR reporting checklist (available at http://dx.doi.org/10.21037/jhmhp-20-25).
Methods
Theoretical framework
Health facilities (denoted as i = 1, 2, …, N) are considered as production entities which transform health inputs (denoted as Xij) into health output (denoted as Yi) (11,12). Health workers, medical supplies, and financial resources are among the input indicators (denoted as j = 1,2, …, K). Similarly, inpatient and outpatient visits are health output indicators (13). TE is one of the indicators used to evaluate the health production performance of health facilities (14-16).
TE of health facilities can be estimated either using Data Envelopment Analysis (DEA) or SFA approach (10,17,18). DEA is a mathematical programming approach which is used to construct efficient production frontiers from input and output combinations of best performing facilities. Any production deviation from the frontier are attributed to technical inefficiency (denoted as ui) (19). This means that DEA does not account for random noise (denoted as vi) which may contribute to production deviations from the frontier (18).
SFA is an econometric approach which is used to estimate efficient production frontiers from input and output combinations accounting for both ui and vi (10). In addition, SFA allows to account for facility heterogeneity (e.g. , facility type, location, and type of management) in the health production model (denoted as zij) (4,9). Due to these merits we employed the SFA approach.
The health production model within the SFA framework can be specified as (20):
[1]
where yi is the health output of facility i; f (. ) is a health production function; xij is health input j of facility i; β denotes a (K ×1) vector of unknown parameters; e is the base of natural logarithm; vi is the stochastic error term; and ui is the non-negative technical inefficiency factor.
From Eq. [1], the TE of facility i (TEi) can be defined as:
[2]
where 0< TEi ≤1. TE is close to zero (or 0%) shows poor performance and TE level close to 1 (or 100%) indicates best performance. The parameters in Eq. [1] can be estimated using maximum likelihood estimation method and ui can be derived using the Jondrow et al. method (4).
The SFA model in Eq. [1] needs to specify a functional form and distribution for ui. We used the Cobb-Douglas (CD) and Translog (TL) functional forms, which are widely used in health care production frontier analysis (17,21). We employed a generalized maximum likelihood-ratio test to select the best functional form as they are nested models (22,23). The commonly assumed ui distributions include half-normal (HN), exponential-normal (EN) and truncated normal (TN) (9). We used the Akaike’s Information Criterion (AIC) and the Bayesian Information Criterion (BIC), which are used to select the most appropriate distribution among non-nested models (24-26) to identify the best distribution of ui.
Empirical model specification
The CD functional form of the SFA model is defined as:
[3]
The TL functional form of the SFA model is defined as:
[4]
where yi refers to health output, xij health input, and zij covariates.
Variable definition
The output, input and covariate variables are presented in Table 1. The selection of output, input, and covariate variables was guided by the purpose of the study, literature, and data availability (16).
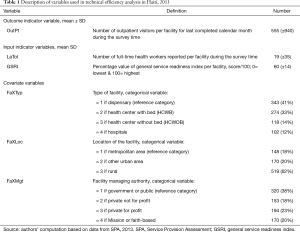
Full table
- Outpatient visit (OutPt) was used as output measure (yi). It refers to number of outpatient visitors in the last completed calendar month during the survey time.
- Health workforce (LaTot) was used as input measure (xij). It refers to number of full-time health workers reported per facility during the survey time. It is the summation of generalist, surgical and specialist medical doctors, nurses, midwife, pharmacist, laboratory technologies, radiologists, dentists, and community health workers.
- General service readiness index (GSRI) was used as the other input measure (xij. The GSRI measures the overall capacity of health facilities to provide general health services (27,28). Following WHO’s guideline (27) facility capacity was assed based on five domains: availability of basic amenities, basic equipment, standard precautions for infection prevention, diagnostic capacity and essential medicines. A score is generated per domain based on the number of domain elements present, then the GSRI was computed based on the mean of the five domains (28).
- Type of facility (FaxTyp), management (FaxMgt), and location (FaxLoc) were used as covariates to control for facility heterogeneity.
Data source
We used Haiti’s Service Provision Assessment (SPA) survey data, which were collected between March 2013 and July 2013 by the Institut Haitien de l’Enfance (IHE) (29). The SPA survey data included, among others, types of available health services including outpatient services at health facility level. In addition, it accounted the number of patients who got each service for last completed calendar month during the survey time and the number of health professionals working in the facilities. Furthermore, the survey covered indicators which help to compute facility level general service provision readiness scores (27,28). The data were part of the facility inventory dataset and accessed with permission from the DHS program website (29).
Unit of analysis
The SPA survey covered 905 health facilities including 358 dispensaries, 297 HCWOB, 129 HCWB, and 121 hospitals which include university, regional, community referral, and other hospitals. However, only the 837 facilities including 343 dispensaries, 274 HCWOB, 118 HCWB, and 102 hospitals had complete data and considered as our unit of analysis. The remaining 68 facilities were dropped from our analysis due to missing data. The study was conducted in accordance with the Declaration of Helsinki (as revised in 2013). Our study did not involve human experiments and it is health facility analysis based on secondary data. Therefore, we do not think that the ethical approval applies to our study.
Data analysis
Both descriptive statistics and econometric analysis techniques were employed. The descriptive statistics analysis technique was used to summarize the output, input and control variables. In addition, this technique was used to summarize TE scores and general service readiness scores.
Following the theoretical and empirical frameworks presented in the preceded sub-sections, the SFA, an econometric analysis technique was employed to predict TE scores of health facilities. It has to be noted that the natural log of the number of outpatient visits and health workers were used in the empirical model. As the GSRI was already expressed in percentages, it was used as it is in the empirical model. We used Stata 15 for data analysis and its sfcross command for the SFA (23,30).
Results
Descriptive statistics of outpatient visits, health workers and service readiness
Table 2 presents the descriptive statistics of outpatient visits, health workers and GSRI by health facility characteristics. Dispensaries have the lowest outpatient visits and health workers. The service readiness index also shows that dispensaries have, on average, a little higher than half of the capacity required to provide general health services. As expected, hospitals have the highest number of outpatient visits and health professionals. Furthermore, hospitals have the highest GSRI compared with other health facility types (for detailed summary statistics of GSRI please refer Table S1).
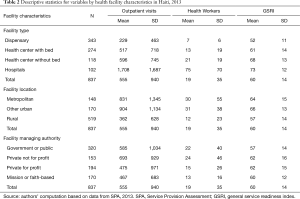
Full table
Health facilities located in urban centers outside the metropolitan area of Port-au-Prince have the highest number of outpatient visitors. They also have the highest number of health workers and are at better capacity to serve general health services compared to the facilities in the other locations. The same trend is observed in health facilities managed by private not for profits. They have the highest number of outpatient visitors and health workers.
SFA results
The first step that we carried out in the SFA was testing whether there is technical inefficiency component in the health production model. Our test statistics show that the null hypothesis of no inefficiency component is rejected (z=−6.59, P<0.000). Accordingly, we proceeded with the use of stochastic frontier model instead of using ordinary least square model.
The second step of our SFA involved in testing whether the CD or TL functional form best fit the health production model assuming ui has TN distribution. Following the one-sided generalized LR test, we fail to reject the null hypothesis which stated the CD model is nested in the TL model {LR chi2 [1] =0.08; P<0.774}. In addition, the CD model’s AIC (i.e. , 2,651) and BIC (i.e. , 2,718) values are relatively smaller than the TL model’s AIC (i.e. , 2,653) and BIC (i.e. , 2,724). However, both functional forms resulted similar estimates to the model parameters and TE scores. Consequently, we presented the CD model results to avoid redundancy.
Finally, we computed the information criteria for the models with HN (AIC =2,655 & BIC =2,716), EN (AIC =2,649 & BIC =2,711), and TN (AIC =2,651 & BIC =2,718) to determine which distribution of the technical inefficiency component to use. The results indicated that the EN distribution has the lowest AIC and BIC values, hence selected for analysis. However, we presented the parameter estimates (Table 3) and TE scores (Table 4) from the three distributions for comparison purpose. We would like also to note that the differences in both AIC and BIC values are relatively marginal (<10) across the three distributions, which makes it hard to choose among the distributions. Furthermore, the CD model with EN and TN distributions resulted similar TE scores. Contrarily, the HN model resulted lower TE scores.
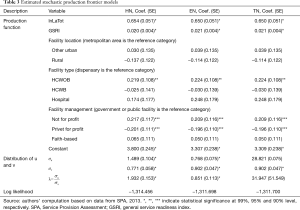
Full table
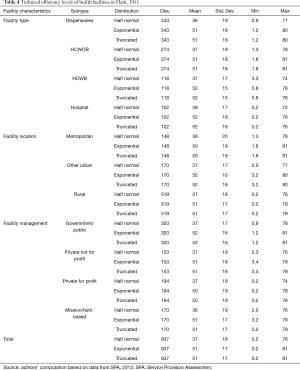
Full table
Association of outpatient visits with health workers and facility service readiness
Table 3 presents the parameter estimates of the SFA model with the CD functional form. The number of health workers and the level of service readiness of health facilities have positive and significant association with outpatient visits. If the number of health workers per health facility increases by 1%, then outpatient visits may increase by 0.65% per month, keeping other factors constant. Similarly, if health facilities increase service readiness, on average, by one percentage point outpatient visits can be increased by up to 2.1%. The results are similar across the three distributions.
TE levels of health facilities in Haiti
Table 4 presents the estimated TE levels disaggregated by basic characteristics of the health facilities. Considering all health facility types, the average TE of health production was estimated at 51% with CD functional form specification and EN distribution. TE with this specification ranges from 0.2% to 81%. However, the average TE falls down to 37%, which range from 0.2% to 78% with CD functional form and HN distribution.
There was little disparity in TE scores when we disaggregate results by various health facility characteristics. The TE of Dispensaries and HCWOB was estimated at 51% whereas that of HCWB and hospitals was 52%. Similarly, health facilities located in non-metropolitan urban centers had relatively higher average TE scores, which was estimated at 52%. Health facilities in rural areas had the second largest average TE score, which was also estimated at 51%. Health facilities in the metropolitan areas had the least TE scores, which was estimated at 50%.
There is also limited variation in TE scores by types of managing authorities of health facilities. Government or public health facilities had relatively higher average TE estimated at 52% followed by private not-for profits and faith-based organizations with average TE estimated at 51%. Private for profits had the lowest TE scores estimated at 50%.
Discussion
In Haiti, outpatient visits are responsive to the number of health workers available per health facility. Our SFA result showed that the elasticity of outpatient visits to health workers is estimated at 0.65%. This means that if the number of health workers per health facility increases by 1%, then outpatient visits may increase by 0.65% per month, keeping other factors constant. A similar study in Ghana (16) reported 0.38% to 0.40% elasticity of outpatient visit with respect to health workers. The positive association between outpatient visits and health workforce in Haiti can be attributed to the gap between health services needs and health workforce shortages. For instance, though WHO recommends 4.45 doctors nurses, and midwives per 1,000 people, Haiti stood at 0.65 only (31). This has to do with problems related to recruitment and allocation of human resource for health (3,32). However, the problem is not only health workforce shortage but also low health workforce productivity. Absenteeism and moonlighting are among the factors for the low productivity (3). This implies that there are unmet health service needs which can be addressed through increasing the availability and productivity of health workforce (33). It is a well-documented fact that availability of health workforce is one of the determinant factors of patients’ healthcare seeking behavior (34). Beyond resolving staff shortages through recruiting new staff, reallocation, and task shifting whenever necessary, promoting results-based financing can help enhance health workforce productivity (3).
General service readiness of health facilities also determines the number of outpatient visits per month in the country. Based on our SFA result, if service readiness is increased by 1%, outpatient visits can be increased by up to 2.1%. This result shows that outpatient visits to health facilities is positively responsive to enhancing health facilities’ capacity to serve general health services in Haiti. As indicated in Table 2, service readiness is the lowest in dispensaries (57%). These are also the facility types which were reported to be with extremely low patient volumes (3). This implies that though readiness does not guarantee quality service provision, it can be said a pre-requisite to deliver basic health services to patients (35,36). However, health facilities in Haiti have, on average, only 60% of the resources required for general health services. For instance, the average diagnostic capacity is 39% and availability of essential medicine is 63%. To get better health outcomes like outpatient visits, the country must increase the readiness of health facilities. A recent study in Haiti (37) found out strong association between readiness and facility-based birth. Therefore, health system reform measures in Haiti must give due emphasis to health facility readiness through enhancing the diagnosis capacity of and availing essential medicine in health facilities.
The key finding of our analysis is the significance potential for TE gains in all types of health facilities in Haiti. Based on the current number of health workers and level of general service readiness, our empirical results indicate that the overall mean TE is estimated at 51%. This indicates that health facilities are serving only a little higher than half of outpatient visits that they would have served. Given the current human resources for health and the general health service capacity, the health facilities can increase outpatient visits by up to 49% if they implement best practices. A similar study in Ghana also reported a TE score of 51% for primary health care facilities (16). However, a previous study in Haiti using DEA technique reported a 92% overall mean TE score (3), which is higher than our finding. This implies that differences in estimation approaches (DEA vs. SFA), and variations in output and input types used leads to TE variations. Therefore, there is a need to carefully consider the appropriate approach which lets to capture statistical noises and consider covariates (38).
However, this study has also a couple of limitations. First, the data we used is for 2013 and the findings may not show the current status of TE of health facilities in Haiti. Second, this analysis is also based on a cross-sectional data and does not show the TE dynamics in the country over time. In addition, the outcome measure is limited to outpatient visits and this can be complemented with other outcome measures. Though the current analysis results give valuable insights they can be improved if latest and panel data can be used for analysis. Furthermore, better insights can also be extracted if service specific readiness with a corresponding outcome measure is used instead of the general service readiness.
Conclusions
The facility-based health production in Haiti is determined by the number of health workers and general service readiness of facilities. Outpatient visit are positively responsive to changes in these inputs, showing the gap in human resources for health and facilities service readiness. The TE of health production was also estimated at 51%, indicating that the health facilities can serve more outpatient visits by up to 49% with the current inputs if they implement best practices. The policy implication of our finding is that countries with limited resources like Haiti can benefit from enhancing the TE of health facilities beyond mobilizing additional resources to improve the health outcomes of their people.
Acknowledgments
Funding: None.
Footnote
Provenance and Peer Review: This article was commissioned by the Guest Editor (Wu Zeng) for the series “Incentives and health system efficiency in low- and middle-income countries” published in Journal of Hospital Management and Health Policy. The article has undergone external peer review.
Reporting Checklist: The authors have completed the MDAR reporting checklist. Available at http://dx.doi.org/10.21037/jhmhp-20-25
Conflicts of Interest: All authors have completed the ICMJE uniform disclosure form (available at http://dx.doi.org/10.21037/jhmhp-20-25). The series “Incentives and health system efficiency in low- and middle-income countries” was commissioned by the editorial office without any funding or sponsorship. The authors have no other conflicts of interest to declare.
Ethical Statement: The authors are accountable for all aspects of the work in ensuring that questions related to the accuracy or integrity of any part of the work are appropriately investigated and resolved. The study was conducted in accordance with the Declaration of Helsinki (as revised in 2013). Our study did not involve human experiments and it is health facility analysis based on secondary data. Therefore, we do not think that the ethical approval applies to our study.
Open Access Statement: This is an Open Access article distributed in accordance with the Creative Commons Attribution-NonCommercial-NoDerivs 4.0 International License (CC BY-NC-ND 4.0), which permits the non-commercial replication and distribution of the article with the strict proviso that no changes or edits are made and the original work is properly cited (including links to both the formal publication through the relevant DOI and the license). See: https://creativecommons.org/licenses/by-nc-nd/4.0/.
References
- Jacobs LD, Judd TM, Bhutta ZA. Addressing the Child and Maternal Mortality Crisis in Haiti through a Central Referral Hospital Providing Countrywide Care. Perm J 2016;20:59-70. [Crossref] [PubMed]
- Lipira L, Kemp C, Domercant JW, et al. The role of service readiness and health care facility factors in attrition from Option B+ in Haiti: a joint examination of electronic medical records and service provision assessment survey data. Int Health 2018;10:54-62. [Crossref] [PubMed]
- Cavagnero ED, Cros MJ, Dunworth AJ, et al. Better spending, better care: a look at Haiti's health financing. The World Bank; 2017.
- Greene W. Distinguishing between heterogeneity and inefficiency: stochastic frontier analysis of the World Health Organization’s panel data on national health care systems. Health Econ 2004;13:959-80. [Crossref] [PubMed]
- Moreno-Serra R, Montes MA, Smith PC. Levels and Determinants of Health System Efficiency in Latin America and the Caribbean. Inter-American Development Bank; 2018. Report No. : 1582.
- World Health Organization (WHO). The World Health Report 2000: health systems: improving performance. Geneva: WHO; 2000.
- Cylus J, Papanicolas I, Smith P. editors. Health System Efficiency: How to make measurement matter for policy and management. Copenhagen, Denmark: WHO Regional Office for Europe; 2016.
- Kalirajan K. Technical Consultation on Measurement of the Efficiency of Health Systems. In: Murray CJ, Evans DB. editors. Health Systems Performance Assessment: Debates, methods and empiricism 2003:109-15.
- Kumbhakar SC, Wang HJ, Horncastle AP. A Practitioner’s Guide to Stochastic Frontier Analysis Using Stata. New York: Cambridge University Press; 2015.
- Lovell K. Frontier Analysis in Healthcare. Int J Healthcare Technology and Management 2006;7:5-14. [Crossref]
- Folland S, Goodman AC, Stano M. The Economics of Health and Health Care. 7 edition. Upper Saddle River, NJ: Prentice Hall 2012:624.
- Peacock S, Chan C, Mangolini M, et al. Techniques for measuring efficiency in health services. Product Comm Staff Work Pap 2001.
- McGlynn EA. Identifying, Categorizing, and Evaluating Health Care Efficiency Measures. Rockville, MD: Agency for Healthcare Research and Quality; 2008. AHRQ Publication No. 08-0030.
- Osei D, d’Almeida S, George MO, et al. Technical efficiency of public district hospitals and health centres in Ghana: a pilot study. Cost Eff Resour Alloc 2005;3:9. [Crossref] [PubMed]
- Zere E, Mbeeli T, Shangula K, et al. Technical efficiency of district hospitals: Evidence from Namibia using Data Envelopment Analysis. Cost Eff Resour Alloc 2006;4:5. [Crossref] [PubMed]
- Novignon J, Nonvignon J. Improving primary health care facility performance in Ghana: efficiency analysis and fiscal space implications. BMC Health Serv Res 2017;17:399. [Crossref] [PubMed]
- Coelli TJ, Rao DSP, O’Donnell CJ, et al. An Introduction to Efficiency and Productivity Analysis 2nd ed. New York, NY: Springer; 2005:349.
- Hollingsworth B. Health system efficiency: measurement and policy. In: Cylus J, Papanicolas I, Smith PC. editors. Health System Efficiency: how to make measurement matter for policy and management. Copenhagen, Denmark: WHO Regional Office for Europe; 2016:99-114.
- Hollingsworth B. The measurement of efficiency and productivity of health care delivery. Health Econ 2008;17:1107-28. [Crossref] [PubMed]
- Aigner D, Lovell K, Schmidt P. Formulation and estimation of stochastic frontier function models. J Econ 1977;6:21-37. [Crossref]
- Hamidi S. Measuring efficiency of governmental hospitals in Palestine using stochastic frontier analysis. Cost Eff Resour Alloc 2016;14:3. [Crossref] [PubMed]
- Greene W. Econometric Analysis. 5th ed. India: Pearson Education; 2003.
- StataCorp. Stata: Release 15. Statistical Software. College Station, TX: StataCorp LLC, 2017.
- Dziak JJ, Coffman DL, Lanza ST, et al. Sensitivity and specificity of information criteria [Internet]. State College, Pennsylvania: College of Health and Human Development, The Pennsylvania State University; 2012. Report No. : Technical Report Series #12-119. Available online: https://www.methodology.psu.edu/files/2019/03/12-119-2e90hc6.pdf
- Vrieze SI. Model selection and psychological theory: A discussion of the differences between the Akaike Information Criterion (AIC) and the Bayesian Information Criterion (BIC). Psychol Methods 2012;17:228-43. [Crossref] [PubMed]
- Lordan G. Measuring Efficiency in Healthcare: An Application to Out of Hours Primary Care Services on the Island of Ireland. Dublin, Ireland: Health Economics and Data Group, The University of York. ; 2007. Report No. : HEDG Working Paper 07/08.
- World Health Organization. Service Availability and Readiness Assessment (SARA): An annual monitoring system for service delivery: Reference Manual 2013.
- World Health Organization. Service Availability and Readiness Assessment (SARA): An annual monitoring system for service delivery: Implementation Guide 2013.
- Institut Haïtien de l’Enfance (IHE), ICF International. Évaluation de Prestation des Services de Soins de Santé, Haïti, 2013 [Dataset]. HTFC6AFLSR.DTA. [Internet]. Rockville, Maryland, USA: IHE et ICF International; 2014. Available online: https://www.dhsprogram.com/pubs/pdf/SPA19/SPA19.pdf
- Belotti F, Daidone S, Ilardi G, et al. Stochastic frontier analysis using Stata. The Stata Journal 2013;13:719-58. [Crossref]
- Abt Associates. Haiti Health Workforce Data Audits Lead to Human Resources for Health Improvements. North Bethesda, MD: Abt Associates 2017.
- Hashimoto K, Adrien L, Rajkumar S. Moving Towards Universal Health Coverage in Haiti. Health Syst Reform 2020;6:e1719339. [Crossref] [PubMed]
- Peragallo Urrutia R, Merisier D, Small M, et al. Unmet health needs identified by Haitian women as priorities for attention: a qualitative study. Reprod Health Matters 2012;20:93-103. [Crossref] [PubMed]
- Jin Y, Zhu W, Yuan B, et al. Impact of health workforce availability on health care seeking behavior of patients with diabetes mellitus in China. Int J Equity Health 2017;16:80. [Crossref] [PubMed]
- Leslie HH, Spiegelman D, Zhou X, et al. Service readiness of health facilities in Bangladesh, Haiti, Kenya, Malawi, Namibia, Nepal, Rwanda, Senegal, Uganda and the United Republic of Tanzania. Bull World Health Org 2017;95:738. [Crossref] [PubMed]
- Wang W, Winner M, Burgert-Brucker CR. Limited service availability, readiness, and use of Facility-Based delivery care in Haiti: a study linking health facility data and population data. Glob Health Sci Pract 2017;5:244-60. [Crossref] [PubMed]
- Kemp CG, Sorensen R, Puttkammer N, et al. Health facility readiness and facility-based birth in Haiti: a maximum likelihood approach to linking household and facility data. J Glob Health Rep 2018;2:e2018023. [Crossref] [PubMed]
- Katharakis G, Katharaki M, Katostaras T. SFA vs. DEA for Measuring Healthcare Efficiency: A Systematic Review. Int J Stat Med Res 2013;2:152-66. [Crossref]
Cite this article as: Kasa L, Bowser D, Nandakumar A. Technical efficiency analysis of health facilities in Haiti: a stochastic frontier approach. J Hosp Manag Health Policy 2021;5:1.